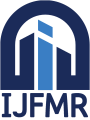
International Journal For Multidisciplinary Research
E-ISSN: 2582-2160
•
Impact Factor: 9.24
A Widely Indexed Open Access Peer Reviewed Multidisciplinary Bi-monthly Scholarly International Journal
Home
Research Paper
Submit Research Paper
Publication Guidelines
Publication Charges
Upload Documents
Track Status / Pay Fees / Download Publication Certi.
Editors & Reviewers
View All
Join as a Reviewer
Reviewer Referral Program
Get Membership Certificate
Current Issue
Publication Archive
Conference
Publishing Conf. with IJFMR
Upcoming Conference(s) ↓
WSMCDD-2025
GSMCDD-2025
Conferences Published ↓
RBS:RH-COVID-19 (2023)
ICMRS'23
PIPRDA-2023
Contact Us
Plagiarism is checked by the leading plagiarism checker
Call for Paper
Volume 6 Issue 6
November-December 2024
Indexing Partners






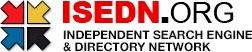

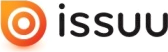


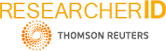
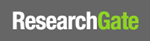
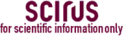
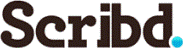

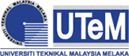
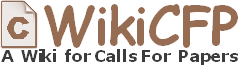
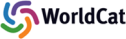
A Comparative Analysis Of Collaborative Filtering Models For Game Recommendation Using Cosine Similarity, SVD, K-Means Clustering And Real-Time Game Insights
Author(s) | Vijay Krishna Sundaran S, Prawin K, Srilakshan A |
---|---|
Country | India |
Abstract | This paper presents a study on GameRS, a game recommendation system that employs collaborative filtering techniques, including Cosine Similarity, Singular Value Decomposition (SVD), and K-means. Clustering, in conjunction with real-time game insights facilitated by Groq AI. The system integrates data from the RAWG API to provide game recommendations and dynamically retrieves game details, including genres, platforms, reviews, ratings, release dates, trailers, and gameplay mechanics. Furthermore, it presents a novel User Satisfaction Index for Games (USIG), a metric designed to assess anticipated enjoyment by considering factors such as rating, genre similarity, and platform similarity. Users may pose specific inquiries related to games, to which Groq AI provides succinct and accurate answers. GameRS, developed with Streamlit, manages both the front-end interface and back-end logic, allowing users to access recommendations, game details, trailers, and additional features. Evaluation results indicate that Cosine Similarity surpasses alternative methods regarding recall and hit rate, whereas SVD and K-means provide insights into latent user preferences and clustering behaviors. |
Keywords | Collaborative Filtering, Cosine Similarity, Singular Value Decomposition, K-means Clustering, Game Recommendation, RAWG API, Streamlit, Groq AI, USIG |
Field | Computer > Artificial Intelligence / Simulation / Virtual Reality |
Published In | Volume 6, Issue 5, September-October 2024 |
Published On | 2024-10-08 |
Cite This | A Comparative Analysis Of Collaborative Filtering Models For Game Recommendation Using Cosine Similarity, SVD, K-Means Clustering And Real-Time Game Insights - Vijay Krishna Sundaran S, Prawin K, Srilakshan A - IJFMR Volume 6, Issue 5, September-October 2024. DOI 10.36948/ijfmr.2024.v06i05.28551 |
DOI | https://doi.org/10.36948/ijfmr.2024.v06i05.28551 |
Short DOI | https://doi.org/g7942x |
Share this

E-ISSN 2582-2160

CrossRef DOI is assigned to each research paper published in our journal.
IJFMR DOI prefix is
10.36948/ijfmr
Downloads
All research papers published on this website are licensed under Creative Commons Attribution-ShareAlike 4.0 International License, and all rights belong to their respective authors/researchers.
