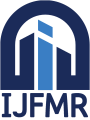
International Journal For Multidisciplinary Research
E-ISSN: 2582-2160
•
Impact Factor: 9.24
A Widely Indexed Open Access Peer Reviewed Multidisciplinary Bi-monthly Scholarly International Journal
Home
Research Paper
Submit Research Paper
Publication Guidelines
Publication Charges
Upload Documents
Track Status / Pay Fees / Download Publication Certi.
Editors & Reviewers
View All
Join as a Reviewer
Get Membership Certificate
Current Issue
Publication Archive
Conference
Publishing Conf. with IJFMR
Upcoming Conference(s) ↓
WSMCDD-2025
GSMCDD-2025
AIMAR-2025
Conferences Published ↓
ICCE (2025)
RBS:RH-COVID-19 (2023)
ICMRS'23
PIPRDA-2023
Contact Us
Plagiarism is checked by the leading plagiarism checker
Call for Paper
Volume 7 Issue 4
July-August 2025
Indexing Partners






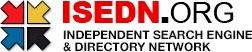

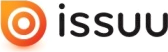


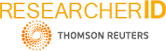
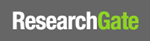
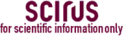
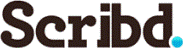

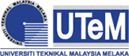
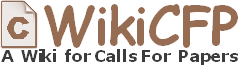
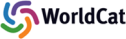
Efficient Ship Detection System for Maritime Surveillance using Deep Learning Approach
Author(s) | Joel Sharon Rubikumar |
---|---|
Country | India |
Abstract | The usage of drones in maritime surveillance is a very effective means to observe ships. As the ship detection is vital for applications such as port monitoring, cross-border surveillance, Moreover, it is not only vital for maritime surveillance but also environmental conservation. Despite its effectiveness, the drone-captured images present itself with its own challenges, specifically when leveraging on a custom dataset which is tailored for specific application. To address the issues, we propose a novel ship detection approach called YOLOv9 with Adan optimizer (YOLOv9-Adan) which is more accurate to increase the efficiency of maritime surveillance. The YOLOv9-Adan model integrates the robust object detection capabilities of the adaptive learning capabilities of the Adan (ADAptive Nesterov momentum algorithm) optimiser, trained on the drone image dataset comprising 3200 images of maritime scenes and ship types in drone view. Our model is trained on a drone-image dataset comprising 3200 images of maritime scenes and ship types in drone views collected from various sources. The experimental results show that our approach using the YOLOv9-Adan model achieves 65.5% mAP, which exceeds the mAP of YOLOv9 by 4.3%. Additionally, This article also provides a comparative analysis of our model YOLOv9-Adan with other existing models in literature with consistently surpassing existing approaches |
Keywords | Ship Detection, Deep Learning, YOLO (You Only Look Once), Adan (ADAptive Nesterov momentum algorithm), PGI(Programmable Gradient Information), GELAN(Generalized Efficient Layer Aggregate Network) |
Field | Computer > Artificial Intelligence / Simulation / Virtual Reality |
Published In | Volume 6, Issue 5, September-October 2024 |
Published On | 2024-10-12 |
DOI | https://doi.org/10.36948/ijfmr.2024.v06i05.28687 |
Short DOI | https://doi.org/g794xw |
Share this

E-ISSN 2582-2160

CrossRef DOI is assigned to each research paper published in our journal.
IJFMR DOI prefix is
10.36948/ijfmr
Downloads
All research papers published on this website are licensed under Creative Commons Attribution-ShareAlike 4.0 International License, and all rights belong to their respective authors/researchers.
