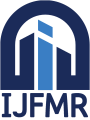
International Journal For Multidisciplinary Research
E-ISSN: 2582-2160
•
Impact Factor: 9.24
A Widely Indexed Open Access Peer Reviewed Multidisciplinary Bi-monthly Scholarly International Journal
Home
Research Paper
Submit Research Paper
Publication Guidelines
Publication Charges
Upload Documents
Track Status / Pay Fees / Download Publication Certi.
Editors & Reviewers
View All
Join as a Reviewer
Get Membership Certificate
Current Issue
Publication Archive
Conference
Publishing Conf. with IJFMR
Upcoming Conference(s) ↓
WSMCDD-2025
GSMCDD-2025
Conferences Published ↓
RBS:RH-COVID-19 (2023)
ICMRS'23
PIPRDA-2023
Contact Us
Plagiarism is checked by the leading plagiarism checker
Call for Paper
Volume 7 Issue 2
March-April 2025
Indexing Partners






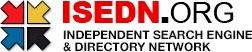

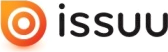


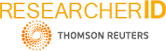
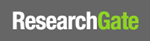
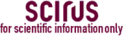
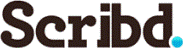

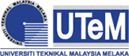
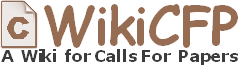
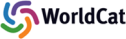
Comparative Analysis of Neural Network Architectures for Automated Fracture Detection in Hand X-ray Images
Author(s) | Jie Zhang, Hongzhen Chen |
---|---|
Country | Thailand |
Abstract | The application of several neural network architectures—including Fully Connected Networks (FCN), Convolutional Neural Networks (CNN), pretrained ResNet, Vision Transformer (ViT-B-16)—for the classification of hand X-ray images into "Fractured" and "Not Fractured"—categories is investigated in this work. The main goals are to evaluate these models' fracture detection ability and determine which architectural design fits this work. Because transfer learning let the model use past information from big-scale picture datasets, the pretrained ResNet model emerged as the most effective with high accuracy, stability, and resilience. The bespoke CNN also performed well, displaying excellent feature extraction powers especially for medical imaging. But the non-pretrained ResNet model overfitted, meaning deeper networks find it difficult to generalize without pretraining. Though innovative, the Vision Transformer performed poorly since it depends on a lot of training data and finds difficult learning of intricate spatial properties from little datasets. Although acting as a baseline, the FCN's simple architecture and incapacity to detect spatial hierarchies in images meant it could not match the efficacy of CNN models. Emphasizing the important function of transfer learning in clinical applications, the results show that pretrained CNN architectures, especially ResNet, offer the most consistent and accurate method for automatic fracture diagnosis in medical pictures. |
Field | Computer > Artificial Intelligence / Simulation / Virtual Reality |
Published In | Volume 6, Issue 5, September-October 2024 |
Published On | 2024-10-20 |
DOI | https://doi.org/10.36948/ijfmr.2024.v06i05.28796 |
Short DOI | https://doi.org/g8np9z |
Share this

E-ISSN 2582-2160

CrossRef DOI is assigned to each research paper published in our journal.
IJFMR DOI prefix is
10.36948/ijfmr
Downloads
All research papers published on this website are licensed under Creative Commons Attribution-ShareAlike 4.0 International License, and all rights belong to their respective authors/researchers.
