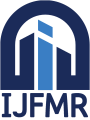
International Journal For Multidisciplinary Research
E-ISSN: 2582-2160
•
Impact Factor: 9.24
A Widely Indexed Open Access Peer Reviewed Multidisciplinary Bi-monthly Scholarly International Journal
Home
Research Paper
Submit Research Paper
Publication Guidelines
Publication Charges
Upload Documents
Track Status / Pay Fees / Download Publication Certi.
Editors & Reviewers
View All
Join as a Reviewer
Get Membership Certificate
Current Issue
Publication Archive
Conference
Publishing Conf. with IJFMR
Upcoming Conference(s) ↓
WSMCDD-2025
GSMCDD-2025
AIMAR-2025
Conferences Published ↓
ICCE (2025)
RBS:RH-COVID-19 (2023)
ICMRS'23
PIPRDA-2023
Contact Us
Plagiarism is checked by the leading plagiarism checker
Call for Paper
Volume 7 Issue 4
July-August 2025
Indexing Partners






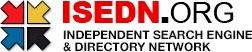

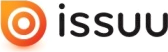


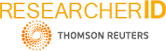
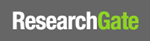
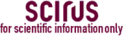
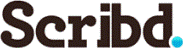

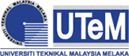
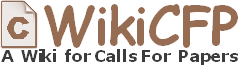
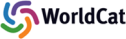
On the Knowledge of Neural Networks: The Genesis, Characterization, and Justification
Author(s) | Huikang Jiang |
---|---|
Country | China |
Abstract | This paper systematically analyzes the knowledge generation process of neural networks through the lens of Philosophy for Science and Epistemology. This paper first argues that for neural network’s knowledge to be possible, it is necessary that the truth of the world can be represented by mathematics, since neural networks are, by their first nature, a mathematical model, and this paper provides few arguments that support the mathematical nature of truth. For a central characterization of neural network’s knowledge, this paper argues that neural networks bypass the three inherent limits of traditional sciences, such as physics and chemistry, suggested by Eugene P. Wigner (1995). The three limits are: 1) Traditional sciences are inevitably approximations of the transcendental truth; 2) Scientists will never stop pursuing deeper, more profound scientific theories that encompass more information than previous theories, despite every theory being an approximation; 3) The increasing complexity and depth of the successive scientific theories poses a significant challenge to human intellect. Neural networks bypass these limits by adaptively learning any underlying function to the given data space, without creating any fundamental hypothesis, which are inevitably not transcendental, like that of traditional sciences. This is also another distinction that supports the differentiation between “machine knowledge” and “human knowledge”, as first proposed by Wheller (2016). This paper argues that the only way humans can justify machine knowledge is through Reliabilism, the epistemic justification through trust in the knowledge generation process. Although reliabilism may currently seem unacceptable, this paper predicts that scientists will take control over machine knowledge in the future, as testified by scientists exploring the chemical world, which is similarly beyond direct comprehension. In the end, this paper suggests the necessity of discussing how AI can justify its own knowledge as conscious beings. |
Keywords | Epistemology, Philosophy for Science, Artificial Intelligence, Neural Networks |
Field | Sociology > Philosophy / Psychology / Religion |
Published In | Volume 6, Issue 5, September-October 2024 |
Published On | 2024-10-22 |
DOI | https://doi.org/10.36948/ijfmr.2024.v06i05.29195 |
Short DOI | https://doi.org/g8pnn2 |
Share this

E-ISSN 2582-2160

CrossRef DOI is assigned to each research paper published in our journal.
IJFMR DOI prefix is
10.36948/ijfmr
Downloads
All research papers published on this website are licensed under Creative Commons Attribution-ShareAlike 4.0 International License, and all rights belong to their respective authors/researchers.
