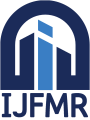
International Journal For Multidisciplinary Research
E-ISSN: 2582-2160
•
Impact Factor: 9.24
A Widely Indexed Open Access Peer Reviewed Multidisciplinary Bi-monthly Scholarly International Journal
Home
Research Paper
Submit Research Paper
Publication Guidelines
Publication Charges
Upload Documents
Track Status / Pay Fees / Download Publication Certi.
Editors & Reviewers
View All
Join as a Reviewer
Get Membership Certificate
Current Issue
Publication Archive
Conference
Publishing Conf. with IJFMR
Upcoming Conference(s) ↓
WSMCDD-2025
GSMCDD-2025
AIMAR-2025
Conferences Published ↓
ICCE (2025)
RBS:RH-COVID-19 (2023)
ICMRS'23
PIPRDA-2023
Contact Us
Plagiarism is checked by the leading plagiarism checker
Call for Paper
Volume 7 Issue 4
July-August 2025
Indexing Partners






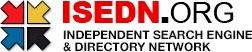

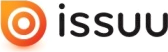


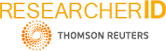
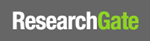
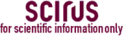
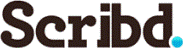

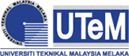
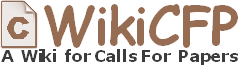
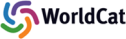
Review on Heart Disease Prediction using Machine Learning Approaches
Author(s) | Runi Ghosh, Nayoneeka Paul, Reba George, Siddharth Das, Shrey Jain, Dr. K Pradeep, Dr K. P. Vijayakumar |
---|---|
Country | India |
Abstract | In this paper, the five machine learning models of Random Forest, Support Vector Machine, Logistic Regression, XGBoost, and K-Nearest Neighbors are carefully compared and evaluated for the prediction of heart disease based on a clinical dataset. The objective is to describe the accuracy, strengths, weaknesses, and applicability of each model with emphasis on which one among the algorithms works best for heart disease diagnosis. We mainly evaluated them in terms of accuracy: Random Forest was the highest among all models, with an accuracy of 89%. It showed good precision and recall abilities owing to being based on ensemble learning. Closely followed was XGBoost with an accuracy of 85% but much more computationally intense, which meant it needed more machine learning processes to compensate for complex clicked patterns. Logistic regression obtained an accuracy of 81%, providing good confidence in recalling positive cases and therefore can identify true-positive instances well. Moderate accuracy at 74% is reported for SVM but its computational intensity and sensitivity to parameter tuning impeded performance. The KNN, with its straightforward design, had lower overall performance, at only 69% accuracy, given that it struggles on feature scaling and is sensitive to irrelevant information in the dataset. Overall, Random Forest and XGBoost have shown the greatest promise in predicting heart disease. Further tuning on these models would enhance their predictive performance in the pursuit of prediction applications. |
Keywords | Heart Disease Prediction, Machine Learning Models, Accuracy Comparison, Clinical Dataset, Model Interpretability, Feature Scaling, Computational Complexity |
Field | Computer > Artificial Intelligence / Simulation / Virtual Reality |
Published In | Volume 6, Issue 5, September-October 2024 |
Published On | 2024-10-29 |
DOI | https://doi.org/10.36948/ijfmr.2024.v06i05.29475 |
Short DOI | https://doi.org/g8qfrg |
Share this

E-ISSN 2582-2160

CrossRef DOI is assigned to each research paper published in our journal.
IJFMR DOI prefix is
10.36948/ijfmr
Downloads
All research papers published on this website are licensed under Creative Commons Attribution-ShareAlike 4.0 International License, and all rights belong to their respective authors/researchers.
