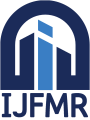
International Journal For Multidisciplinary Research
E-ISSN: 2582-2160
•
Impact Factor: 9.24
A Widely Indexed Open Access Peer Reviewed Multidisciplinary Bi-monthly Scholarly International Journal
Home
Research Paper
Submit Research Paper
Publication Guidelines
Publication Charges
Upload Documents
Track Status / Pay Fees / Download Publication Certi.
Editors & Reviewers
View All
Join as a Reviewer
Reviewer Referral Program
Get Membership Certificate
Current Issue
Publication Archive
Conference
Publishing Conf. with IJFMR
Upcoming Conference(s) ↓
WSMCDD-2025
GSMCDD-2025
Conferences Published ↓
RBS:RH-COVID-19 (2023)
ICMRS'23
PIPRDA-2023
Contact Us
Plagiarism is checked by the leading plagiarism checker
Call for Paper
Volume 6 Issue 6
November-December 2024
Indexing Partners






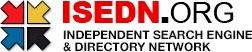

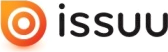


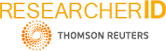
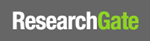
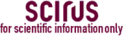
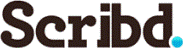

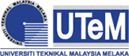
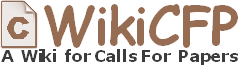
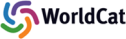
Integrated Deep Learning Architectures for Perception, Control, and Decision-making in Robotics: a Framework for Sensing, Cognition, and Transparent Decision-making
Author(s) | Ruchik Kashyapkumar Thaker |
---|---|
Country | Canada |
Abstract | The increasing complexity of robotic applications demands innovative approaches for addressing problems that lack analytical solutions, with deep learning (DL) emerging as a key tool for enabling robots to learn and adapt in dynamic environments. This survey reviews existing DL techniques in robotics, categorizing the major challenges and exploring successful solutions that leverage DL for perception, control, and decision-making. We discuss the use of modular versus end-to-end DL architectures, providing guidelines for selecting appropriate model structures and training strategies. The review also examines advancements in neuro-robotics systems (NRS), where the convergence of neuroscience and robotics is driving the development of robots with embodied intelligence, enabling more natural human-robot interactions. Recent progress in neural mechanisms for perception, cognition, learning, and control is highlighted, offering insights into creating future neuro-robots. Furthermore, we address the challenge of uncertainty estimation in neural networks, crucial for reliable robotic decision-making, and evaluate existing frameworks such as Bayesian belief networks and Monte Carlo sampling that improve uncertainty modeling without requiring architectural changes. Lastly, we explore efforts to enhance transparency in robotic systems through integrated reasoning and learning methods, focusing on architectures that combine logical reasoning with deep learning to provide explainable decision-making. This survey aims to offer a structured overview of current research and guide future developments in neuro-robotics. |
Keywords | Deep learning (DL), Robotics, Perception, Control, Decision-making, Neuro-robotics systems (NRS), Embodied intelligence, Neural networks |
Field | Computer > Automation / Robotics |
Published In | Volume 6, Issue 5, September-October 2024 |
Published On | 2024-10-03 |
Cite This | Integrated Deep Learning Architectures for Perception, Control, and Decision-making in Robotics: a Framework for Sensing, Cognition, and Transparent Decision-making - Ruchik Kashyapkumar Thaker - IJFMR Volume 6, Issue 5, September-October 2024. DOI 10.36948/ijfmr.2024.v06i05.29708 |
DOI | https://doi.org/10.36948/ijfmr.2024.v06i05.29708 |
Short DOI | https://doi.org/g8pnjc |
Share this

E-ISSN 2582-2160

CrossRef DOI is assigned to each research paper published in our journal.
IJFMR DOI prefix is
10.36948/ijfmr
Downloads
All research papers published on this website are licensed under Creative Commons Attribution-ShareAlike 4.0 International License, and all rights belong to their respective authors/researchers.
