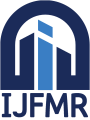
International Journal For Multidisciplinary Research
E-ISSN: 2582-2160
•
Impact Factor: 9.24
A Widely Indexed Open Access Peer Reviewed Multidisciplinary Bi-monthly Scholarly International Journal
Home
Research Paper
Submit Research Paper
Publication Guidelines
Publication Charges
Upload Documents
Track Status / Pay Fees / Download Publication Certi.
Editors & Reviewers
View All
Join as a Reviewer
Get Membership Certificate
Current Issue
Publication Archive
Conference
Publishing Conf. with IJFMR
Upcoming Conference(s) ↓
WSMCDD-2025
GSMCDD-2025
Conferences Published ↓
RBS:RH-COVID-19 (2023)
ICMRS'23
PIPRDA-2023
Contact Us
Plagiarism is checked by the leading plagiarism checker
Call for Paper
Volume 7 Issue 2
March-April 2025
Indexing Partners






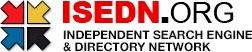

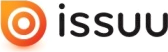


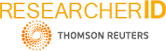
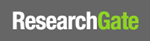
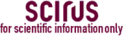
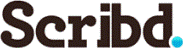

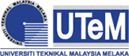
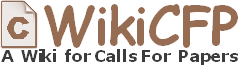
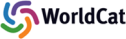
Machine Learning & Deep Learning: Classifying Disaster Tweets Methods
Author(s) | Rohan Nadekar, Saif Kumbay, Jayesh Walke, Najim tadvi, Arnav Mone, Dr Manisha Mali |
---|---|
Country | India |
Abstract | With the increasing reliance on social media sites, and more importantly on Twitter, during disaster events, there is a strong need for an immediate capability to effectively analyze large quantities of near-real-time data into meaningful information in support of more efficient emergency responses. This paper reviews new trends in disaster tweet classification using machine learning and deep learning. We have picked five major studies with approaches ranging from more basic approaches to more complex approaches with Logistic Regression and Naive Bayes to using CNN and BERT deep architectures. Findings of this study include apparent flips in the use of BERT embeddings for improving accuracy and relevance of disaster-related tweet classification, which essentially provides emergency responders with timely and critical information when crises arise.. Review work calls for the input of advanced deep learning techniques to answer the complexities involved with the real-time data streaming that characterizes a disaster scenario. |
Keywords | Disaster response, tweet classification, machine learning, deep learning, BERT embeddings, social media analytics |
Field | Engineering |
Published In | Volume 6, Issue 6, November-December 2024 |
Published On | 2024-11-25 |
DOI | https://doi.org/10.36948/ijfmr.2024.v06i06.29975 |
Short DOI | https://doi.org/g8r8k6 |
Share this

E-ISSN 2582-2160

CrossRef DOI is assigned to each research paper published in our journal.
IJFMR DOI prefix is
10.36948/ijfmr
Downloads
All research papers published on this website are licensed under Creative Commons Attribution-ShareAlike 4.0 International License, and all rights belong to their respective authors/researchers.
