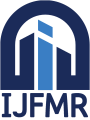
International Journal For Multidisciplinary Research
E-ISSN: 2582-2160
•
Impact Factor: 9.24
A Widely Indexed Open Access Peer Reviewed Multidisciplinary Bi-monthly Scholarly International Journal
Home
Research Paper
Submit Research Paper
Publication Guidelines
Publication Charges
Upload Documents
Track Status / Pay Fees / Download Publication Certi.
Editors & Reviewers
View All
Join as a Reviewer
Get Membership Certificate
Current Issue
Publication Archive
Conference
Publishing Conf. with IJFMR
Upcoming Conference(s) ↓
WSMCDD-2025
GSMCDD-2025
Conferences Published ↓
RBS:RH-COVID-19 (2023)
ICMRS'23
PIPRDA-2023
Contact Us
Plagiarism is checked by the leading plagiarism checker
Call for Paper
Volume 7 Issue 2
March-April 2025
Indexing Partners






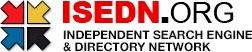

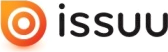


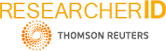
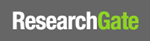
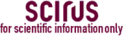
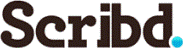

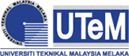
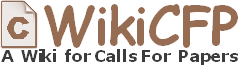
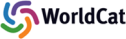
Explainable Artificial Intelligence (XAI) in Healthcare: Enhancing Transparency and Trust
Author(s) | Jaishankar Inukonda, Vidya Rajasekhara Reddy Tetala, Jayanna Hallur |
---|---|
Country | United States |
Abstract | Artificial Intelligence has now taken a full-fledged role in healthcare and has started driving innovations not only in diagnostics and treatment planning but also in patient monitoring and operational efficiency. This will enable complex medical data analysis, extracting patterns and insights that no human is capable of. However, most of these models are per se opaque-that is, the so-called "black-box" problem-there are still great challenges in areas such as transparency, trust, and ethical applications in a clinical setting. This lack of interpretability can stand in the way of acceptance or integration for AI technologies when issues of understanding and accountability are relevant. Explainable AI solves these problems by making real artificial intelligence decisions understand the decisions made to humans. XAI techniques offer well-understandable and interpretable explanations of the models with minimum degradations in performance. This review article explains in detail the critical role of XAI in healthcare, underpinning how this field can bring more transparency into AI applications. We explain some of the current methods of XAI: model-agnostic techniques like LIME and SHAP, interpretable models relating to decision trees and linear models, and visualization techniques like saliency maps and mechanisms of attention. |
Keywords | Explainable Artificial Intelligence (XAI), Healthcare, Transparency, Trust, AI Model Interpretability, Clinical Decision-Making, Ethical AI, Medical Imaging, Electronic Health Records (EHR), Patient Engagement, Digital Healthcare |
Field | Computer Applications |
Published In | Volume 6, Issue 6, November-December 2024 |
Published On | 2024-11-06 |
DOI | https://doi.org/10.36948/ijfmr.2024.v06i06.30010 |
Short DOI | https://doi.org/g8qftx |
Share this

E-ISSN 2582-2160

CrossRef DOI is assigned to each research paper published in our journal.
IJFMR DOI prefix is
10.36948/ijfmr
Downloads
All research papers published on this website are licensed under Creative Commons Attribution-ShareAlike 4.0 International License, and all rights belong to their respective authors/researchers.
