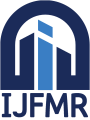
International Journal For Multidisciplinary Research
E-ISSN: 2582-2160
•
Impact Factor: 9.24
A Widely Indexed Open Access Peer Reviewed Multidisciplinary Bi-monthly Scholarly International Journal
Home
Research Paper
Submit Research Paper
Publication Guidelines
Publication Charges
Upload Documents
Track Status / Pay Fees / Download Publication Certi.
Editors & Reviewers
View All
Join as a Reviewer
Reviewer Referral Program
Get Membership Certificate
Current Issue
Publication Archive
Conference
Publishing Conf. with IJFMR
Upcoming Conference(s) ↓
WSMCDD-2025
GSMCDD-2025
Conferences Published ↓
RBS:RH-COVID-19 (2023)
ICMRS'23
PIPRDA-2023
Contact Us
Plagiarism is checked by the leading plagiarism checker
Call for Paper
Volume 6 Issue 6
November-December 2024
Indexing Partners






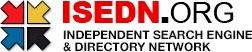

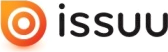


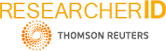
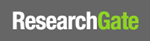
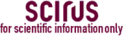
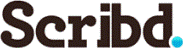

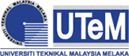
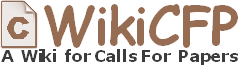
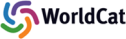
A Comparative Framework for Intent Classification Systems: Evaluating Large Language Models versus Traditional Machine Learning in Contact Center Applications
Author(s) | Santhosh Kumar Ganesan |
---|---|
Country | United States |
Abstract | Modern contact centers face increasingly complex decisions when selecting appropriate technologies for intent identification systems. This article presents a systematic comparative analysis of Large Language Models (LLMs) and traditional Machine Learning (ML) approaches in contact center environments, examining their relative efficacy across various operational contexts. Through a comprehensive evaluation framework, we assess seven critical dimensions: data complexity, training requirements, performance metrics, resource utilization, customization capabilities, deployment considerations, and hybrid implementation strategies. Our findings indicate that LLMs demonstrate superior performance in scenarios involving complex linguistic patterns and contextual understanding, while traditional ML models maintain advantages in resource-constrained environments and clearly defined intent categories. We propose a novel decision framework that enables organizations to optimize their technology selection based on specific operational requirements, resource availability, and performance needs. The article contributes to both theoretical understanding and practical implementation by providing evidence-based guidelines for selecting and implementing intent identification systems. The results suggest that hybrid approaches, combining the strengths of both LLMs and traditional ML models, offer promising solutions for organizations seeking to balance sophisticated language understanding with operational efficiency These findings have significant implications for contact center automation strategies and provide a foundation for future research in adaptive intent classification systems. |
Keywords | Keywords: Intent Identification Systems, Large Language Models (LLMs), Contact Center Automation, Machine Learning Classification, Natural Language Processing. |
Field | Computer |
Published In | Volume 6, Issue 6, November-December 2024 |
Published On | 2024-11-15 |
Cite This | A Comparative Framework for Intent Classification Systems: Evaluating Large Language Models versus Traditional Machine Learning in Contact Center Applications - Santhosh Kumar Ganesan - IJFMR Volume 6, Issue 6, November-December 2024. DOI 10.36948/ijfmr.2024.v06i06.30430 |
DOI | https://doi.org/10.36948/ijfmr.2024.v06i06.30430 |
Short DOI | https://doi.org/g8rd4m |
Share this

E-ISSN 2582-2160

CrossRef DOI is assigned to each research paper published in our journal.
IJFMR DOI prefix is
10.36948/ijfmr
Downloads
All research papers published on this website are licensed under Creative Commons Attribution-ShareAlike 4.0 International License, and all rights belong to their respective authors/researchers.
