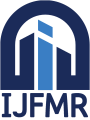
International Journal For Multidisciplinary Research
E-ISSN: 2582-2160
•
Impact Factor: 9.24
A Widely Indexed Open Access Peer Reviewed Multidisciplinary Bi-monthly Scholarly International Journal
Home
Research Paper
Submit Research Paper
Publication Guidelines
Publication Charges
Upload Documents
Track Status / Pay Fees / Download Publication Certi.
Editors & Reviewers
View All
Join as a Reviewer
Reviewer Referral Program
Get Membership Certificate
Current Issue
Publication Archive
Conference
Publishing Conf. with IJFMR
Upcoming Conference(s) ↓
WSMCDD-2025
GSMCDD-2025
Conferences Published ↓
RBS:RH-COVID-19 (2023)
ICMRS'23
PIPRDA-2023
Contact Us
Plagiarism is checked by the leading plagiarism checker
Call for Paper
Volume 6 Issue 6
November-December 2024
Indexing Partners






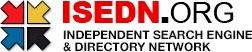

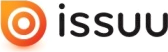


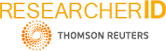
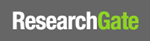
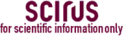
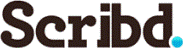

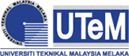
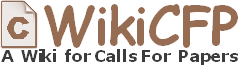
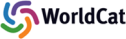
Advancing Hierarchical Model: Evaluating Performance, Interpretability and Implications
Author(s) | Seth Opoku Larbi, Apaka Rangita, Joyce Otieno |
---|---|
Country | Kenya |
Abstract | Hierarchical data structures emerge when observations are nested within higher-level units or clusters. Existing research often ignores the hierarchical structure of data leading to biased estimates, suboptimal model selection, and challenges in identifying important predictors and dependencies. This study aims to contribute to hierarchical frameworks by improving challenges with the interpretability of the random effect, scalability, and computation feasibility in the traditional hierarchical Bayesian model. The authors’ model is an advancement of the Standard hierarchical Bayesian model which introduced a unique variable to the model and parameters to the random effects. The advancements in hierarchical modelling by the authors have significantly improved the accuracy, reliability, and interpretability of the model analysis. Hierarchical Bayesian Information Criteria (HBIC) is the method of selecting variables in the models. The findings of the research indicated that the introduction of Phi (ϕ_j) and Psi (ψ_j) as shrinkage parameters are instrumental in the regulation of parameter estimates towards a common value, resulting in more accurate estimation and less overfitting in the Hierarchical Bayesian Model as well as accounting for the presence of heterogeneity. The introduction of the unique variable (z) allows the model to capture cluster-specific effects associated with the (z). Lastly, the study found that the authors' innovative model outperformed the standard model by improving the accuracy, interpretability, scalability, and regularisation through shrinkage parameters and innovative (z) as the contextual variable. |
Keywords | Shrinkage, Hierarchical, Parameters, Prior, Posterior, Uncertainty, Lasso, Unique Variable |
Field | Mathematics > Statistics |
Published In | Volume 6, Issue 6, November-December 2024 |
Published On | 2024-11-25 |
Cite This | Advancing Hierarchical Model: Evaluating Performance, Interpretability and Implications - Seth Opoku Larbi, Apaka Rangita, Joyce Otieno - IJFMR Volume 6, Issue 6, November-December 2024. DOI 10.36948/ijfmr.2024.v06i06.30796 |
DOI | https://doi.org/10.36948/ijfmr.2024.v06i06.30796 |
Short DOI | https://doi.org/g8r8kv |
Share this

E-ISSN 2582-2160

CrossRef DOI is assigned to each research paper published in our journal.
IJFMR DOI prefix is
10.36948/ijfmr
Downloads
All research papers published on this website are licensed under Creative Commons Attribution-ShareAlike 4.0 International License, and all rights belong to their respective authors/researchers.
