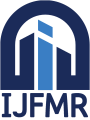
International Journal For Multidisciplinary Research
E-ISSN: 2582-2160
•
Impact Factor: 9.24
A Widely Indexed Open Access Peer Reviewed Multidisciplinary Bi-monthly Scholarly International Journal
Home
Research Paper
Submit Research Paper
Publication Guidelines
Publication Charges
Upload Documents
Track Status / Pay Fees / Download Publication Certi.
Editors & Reviewers
View All
Join as a Reviewer
Get Membership Certificate
Current Issue
Publication Archive
Conference
Publishing Conf. with IJFMR
Upcoming Conference(s) ↓
WSMCDD-2025
GSMCDD-2025
Conferences Published ↓
RBS:RH-COVID-19 (2023)
ICMRS'23
PIPRDA-2023
Contact Us
Plagiarism is checked by the leading plagiarism checker
Call for Paper
Volume 7 Issue 2
March-April 2025
Indexing Partners






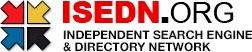

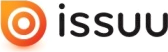


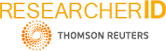
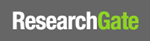
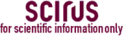
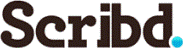

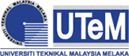
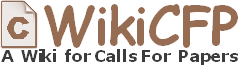
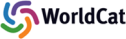
Synthetic Data Augmentation and Deep Learning for Real-Time Weed Detection in Agricultural Fields
Author(s) | M I Nuha Marzuqha, Tshewang Rigzin, Sonam Tharchen |
---|---|
Country | India |
Abstract | The ability to identify weeds in agricultural fields is critical to increasing productivity of crops as well as minimizing the use of herbicides. This paper presents a combined approach focusing on crop-weed discrimination employing the object detection capabilities of YOLOv8, the image classification power of VGG16 and, Grad-CAM, for the purpose of understanding the concerns of the model predictions. With a custom dataset of images consisting of mixed and clean images of potato and carrot crops, enhanced data limitations are achieved by training a CycleGAN model to synthesize clean carrot images from carrot-weed composite images, thus augmenting the dataset and allowing for better model performance in different settings. The pipeline begins with a vision object detection network called YOLOv8, which is used to detect the crops and the weeds in the image by drawing bounding boxes around the areas of interest. VGG16 then takes it a step further by classifying the regions, specifically differentiating between crops and weeds even more accurately. Classification outcomes are further enhanced by Grad-CAM which helps to visualize and elucidate the classifications giving an understanding of an area of interest in the models’ prediction. when assessed across the various metrics, the combined strategy improves the precision and recall measures over the single model systems. This two-model modular design avails a suitable approach for weed detection in the fields in a real-time situation and can be extended for crop monitoring and precision agriculture purposes. |
Keywords | Precision Agriculture, Weed Detection, YOLOv8, VGG16, CycleGAN, Grad-CAM, Crop Management, Object Detection, Image Classification, Machine Learning, Synthetic Data Augmentation. |
Field | Computer > Artificial Intelligence / Simulation / Virtual Reality |
Published In | Volume 6, Issue 6, November-December 2024 |
Published On | 2024-11-25 |
Cite This | Synthetic Data Augmentation and Deep Learning for Real-Time Weed Detection in Agricultural Fields - M I Nuha Marzuqha, Tshewang Rigzin, Sonam Tharchen - IJFMR Volume 6, Issue 6, November-December 2024. DOI 10.36948/ijfmr.2024.v06i06.31555 |
DOI | https://doi.org/10.36948/ijfmr.2024.v06i06.31555 |
Short DOI | https://doi.org/g8r8hb |
Share this

E-ISSN 2582-2160

CrossRef DOI is assigned to each research paper published in our journal.
IJFMR DOI prefix is
10.36948/ijfmr
Downloads
All research papers published on this website are licensed under Creative Commons Attribution-ShareAlike 4.0 International License, and all rights belong to their respective authors/researchers.
