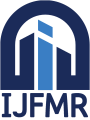
International Journal For Multidisciplinary Research
E-ISSN: 2582-2160
•
Impact Factor: 9.24
A Widely Indexed Open Access Peer Reviewed Multidisciplinary Bi-monthly Scholarly International Journal
Home
Research Paper
Submit Research Paper
Publication Guidelines
Publication Charges
Upload Documents
Track Status / Pay Fees / Download Publication Certi.
Editors & Reviewers
View All
Join as a Reviewer
Get Membership Certificate
Current Issue
Publication Archive
Conference
Publishing Conf. with IJFMR
Upcoming Conference(s) ↓
WSMCDD-2025
GSMCDD-2025
AIMAR-2025
Conferences Published ↓
ICCE (2025)
RBS:RH-COVID-19 (2023)
ICMRS'23
PIPRDA-2023
Contact Us
Plagiarism is checked by the leading plagiarism checker
Call for Paper
Volume 7 Issue 4
July-August 2025
Indexing Partners






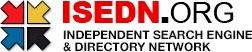

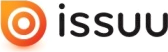


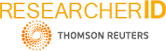
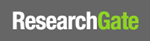
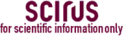
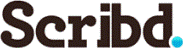

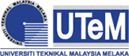
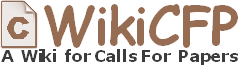
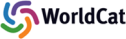
Optimizing Machine Learning Models: A Data Engineering Perspective
Author(s) | Madhukar Dharavath |
---|---|
Country | United States |
Abstract | This thorough article examines how data engineering and machine learning optimization intersect, emphasizing important tactics for improving model performance. It looks at basic topics such as cloud infrastructure scalability, feature engineering, data quality engineering, and hyperparameter optimization. The article illustrates how sound data engineering techniques greatly increase model correctness, lower error rates, and quicken development timelines through the examination of numerous industry applications and research findings. To create effective machine learning systems, the article specifically highlights the significance of automated validation frameworks, domain-specific feature development, methodical optimization techniques, and cloud-native architectures. It illustrates the critical importance of data engineering in attaining superior model performance and offers practitioners practical insights for putting into practice efficient machine learning optimization strategies by looking at real-world applications across several industries. |
Keywords | Data Engineering Optimization, Machine Learning Infrastructure, Feature Engineering Automation, Hyperparameter Tuning, Cloud-Native ML Operations |
Field | Computer |
Published In | Volume 6, Issue 6, November-December 2024 |
Published On | 2024-12-04 |
DOI | https://doi.org/10.36948/ijfmr.2024.v06i06.32242 |
Short DOI | https://doi.org/g8tv8p |
Share this

E-ISSN 2582-2160

CrossRef DOI is assigned to each research paper published in our journal.
IJFMR DOI prefix is
10.36948/ijfmr
Downloads
All research papers published on this website are licensed under Creative Commons Attribution-ShareAlike 4.0 International License, and all rights belong to their respective authors/researchers.
