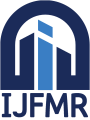
International Journal For Multidisciplinary Research
E-ISSN: 2582-2160
•
Impact Factor: 9.24
A Widely Indexed Open Access Peer Reviewed Multidisciplinary Bi-monthly Scholarly International Journal
Home
Research Paper
Submit Research Paper
Publication Guidelines
Publication Charges
Upload Documents
Track Status / Pay Fees / Download Publication Certi.
Editors & Reviewers
View All
Join as a Reviewer
Get Membership Certificate
Current Issue
Publication Archive
Conference
Publishing Conf. with IJFMR
Upcoming Conference(s) ↓
WSMCDD-2025
GSMCDD-2025
Conferences Published ↓
RBS:RH-COVID-19 (2023)
ICMRS'23
PIPRDA-2023
Contact Us
Plagiarism is checked by the leading plagiarism checker
Call for Paper
Volume 7 Issue 2
March-April 2025
Indexing Partners






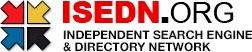

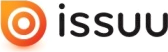


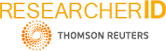
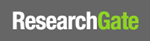
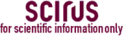
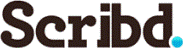

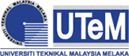
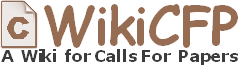
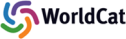
Hierarchical Approaches to Handwritten Digit Recognition: A Study of Modern Neural Networks
Author(s) | Ismail Hossain Sadhin, Elora Majumder Bandhan, Md. Abdullah Al Mamun |
---|---|
Country | Bangladesh |
Abstract | Handwritten digit recognition has several applications in multiple industries in this modern era for enhancing efficiency, accuracy, and accessibility. Convolution Neural Networking (CNNs) have emerged for the precise result of this task since it is a powerful tool for this task due to the ability to learn hierarchical features from data. Several architectures of convolution neural networking (CNNs) are used in this field. This study investigated the efficiency of CNN architectures in recognizing handwritten digits. The considered architectures are VGG16, ResNet50, and DenseNet. However, DenseNet stands out with the highest efficiency of 99.19% compared to traditional architectures like VGG16 and ResNet50. A benchmarked dataset of the machine learning field, MNIST, has been used for training data. Through experimental evaluation, it was observed that the three CNN architectures mentioned achieved high accuracy rates on the MNIST dataset, namely 95.9% for VGG16, 98.5% for ResNet50, and 99.19% for DenseNet. However, DenseNet has been proven to be the most accurate. |
Keywords | Handwritten recognition, Digit recognition, MNIST, Neural network, Machine learning |
Field | Computer > Artificial Intelligence / Simulation / Virtual Reality |
Published In | Volume 6, Issue 6, November-December 2024 |
Published On | 2024-12-10 |
DOI | https://doi.org/10.36948/ijfmr.2024.v06i06.32390 |
Short DOI | https://doi.org/g8vgj2 |
Share this

E-ISSN 2582-2160

CrossRef DOI is assigned to each research paper published in our journal.
IJFMR DOI prefix is
10.36948/ijfmr
Downloads
All research papers published on this website are licensed under Creative Commons Attribution-ShareAlike 4.0 International License, and all rights belong to their respective authors/researchers.
