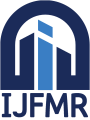
International Journal For Multidisciplinary Research
E-ISSN: 2582-2160
•
Impact Factor: 9.24
A Widely Indexed Open Access Peer Reviewed Multidisciplinary Bi-monthly Scholarly International Journal
Home
Research Paper
Submit Research Paper
Publication Guidelines
Publication Charges
Upload Documents
Track Status / Pay Fees / Download Publication Certi.
Editors & Reviewers
View All
Join as a Reviewer
Get Membership Certificate
Current Issue
Publication Archive
Conference
Publishing Conf. with IJFMR
Upcoming Conference(s) ↓
WSMCDD-2025
GSMCDD-2025
Conferences Published ↓
RBS:RH-COVID-19 (2023)
ICMRS'23
PIPRDA-2023
Contact Us
Plagiarism is checked by the leading plagiarism checker
Call for Paper
Volume 7 Issue 2
March-April 2025
Indexing Partners






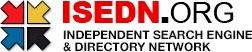

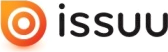


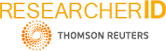
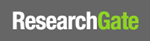
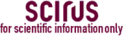
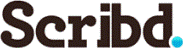

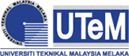
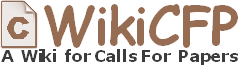
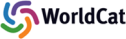
Artificial Intelligence and Machine Learning in Cloud Systems: A Comprehensive Framework for Real-Time Optimization
Author(s) | Varshini Choudary Nuvvula |
---|---|
Country | United States |
Abstract | Abstract This article comprehensively analyzes artificial intelligence and machine learning integration in real-time cloud-based system optimization. The current research and emerging technologies examine how AI-driven algorithms enhance dynamic resource allocation, workload management, and automated decision-making processes in cloud computing environments. The article investigates the implementation of predictive analytics for workload forecasting, machine learning-based anomaly detection, and reinforcement learning approaches for autonomous system optimization. The findings demonstrate significant improvements in resource utilization efficiency, load balancing effectiveness, and system response time compared to traditional rule-based methods. The article also reveals that AI-powered auto-scaling mechanisms substantially enhance cloud system adaptability to varying workload patterns while minimizing operational costs. Moreover, it identifies key challenges in implementing these technologies, including integration complexity and performance overhead considerations, and proposes practical solutions for enterprise adoption. This article contributes to the growing knowledge in cloud computing optimization and provides valuable insights for researchers and practitioners in cloud infrastructure management. |
Keywords | Abstract This article comprehensively analyzes artificial intelligence and machine learning integration in real-time cloud-based system optimization. The current research and emerging technologies examine how AI-driven algorithms enhance dynamic resource allocation, workload management, and automated decision-making processes in cloud computing environments. The article investigates the implementation of predictive analytics for workload forecasting, machine learning-based anomaly detection, and reinforcement learning approaches for autonomous system optimization. The findings demonstrate significant improvements in resource utilization efficiency, load balancing effectiveness, and system response time compared to traditional rule-based methods. The article also reveals that AI-powered auto-scaling mechanisms substantially enhance cloud system adaptability to varying workload patterns while minimizing operational costs. Moreover, it identifies key challenges in implementing these technologies, including integration complexity and performance overhead considerations, and proposes practical solutions for enterprise adoption. This article contributes to the growing knowledge in cloud computing optimization and provides valuable insights for researchers and practitioners in cloud infrastructure management. |
Field | Computer |
Published In | Volume 6, Issue 6, November-December 2024 |
Published On | 2024-12-13 |
DOI | https://doi.org/10.36948/ijfmr.2024.v06i06.32566 |
Short DOI | https://doi.org/g8wkph |
Share this

E-ISSN 2582-2160

CrossRef DOI is assigned to each research paper published in our journal.
IJFMR DOI prefix is
10.36948/ijfmr
Downloads
All research papers published on this website are licensed under Creative Commons Attribution-ShareAlike 4.0 International License, and all rights belong to their respective authors/researchers.
