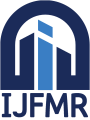
International Journal For Multidisciplinary Research
E-ISSN: 2582-2160
•
Impact Factor: 9.24
A Widely Indexed Open Access Peer Reviewed Multidisciplinary Bi-monthly Scholarly International Journal
Home
Research Paper
Submit Research Paper
Publication Guidelines
Publication Charges
Upload Documents
Track Status / Pay Fees / Download Publication Certi.
Editors & Reviewers
View All
Join as a Reviewer
Get Membership Certificate
Current Issue
Publication Archive
Conference
Publishing Conf. with IJFMR
Upcoming Conference(s) ↓
WSMCDD-2025
GSMCDD-2025
Conferences Published ↓
RBS:RH-COVID-19 (2023)
ICMRS'23
PIPRDA-2023
Contact Us
Plagiarism is checked by the leading plagiarism checker
Call for Paper
Volume 7 Issue 2
March-April 2025
Indexing Partners






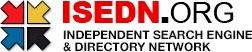

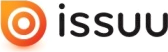


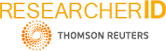
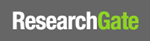
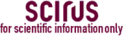
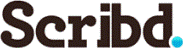

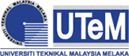
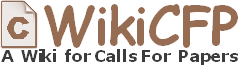
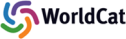
Skin Cancer Detection using CNN (Convolution Neural Network) with AI Medical Assistant
Author(s) | Revathy B D, Rohan Vijay, B Ullas, Sagar N H, Dhyan M |
---|---|
Country | India |
Abstract | Skin cancer is a very common disease, and with numerous morphological forms and characteristics that are similar between malignant and benign skin lesions, makes the diagnosis pretty difficult. Recent trends in deep learning, with CNNs being the first to enter this domain, have highly facilitated the classification and detection of skin cancer, even in its nascent stages, from images. A plethora of such research works proposed novel architectures for CNNs that may possibly improve the accuracy in the diagnosis of the lesion given as against those belonging to seborrheic keratosis, nevus, or melanoma. The best model, according to the works on these authors, was the multi-layer deep learning model known as SkinLesNet, which performed better than established models such as ResNet50 and VGG16 in all datasets. More extensive studies present a DCNN model that addresses the issue of class imbalance and is stronger than transfer learning models, such as DenseNet and MobileNet. This model achieved strong accuracy on several instances: 98.5% with HAM10000 and 97.1% with ISIC-2019 data. Optimizations in model selection methods: In addition, there existed optimizations in model selection. This was through the application of the multiple-criteria decision making approaches. For instance, the method RAPS can be taken as an example. Through the application of this method, a very significant increase in accuracy was achieved. For optimizing the use of feature selection using Inception V3 in determining diagnosis for oral cavity squamous cell carcinoma, BPSO showed an accuracy of 96.3%. Such breakthroughs underpin therefore the capability of deep learning and AI techniques in raising diagnostic accuracy while cutting costs and making a chance for early intervention of skin and other cancers. |
Keywords | Skin cancer, Melanoma, Non-melanoma, CNN, Deep learning, AI, Dermoscopy, Image classification, Machine learning, Early detection, Transfer learning, Feature optimization, HAM10000, ISIC, Medical imaging. |
Field | Computer > Artificial Intelligence / Simulation / Virtual Reality |
Published In | Volume 6, Issue 6, November-December 2024 |
Published On | 2024-12-07 |
DOI | https://doi.org/10.36948/ijfmr.2024.v06i06.32582 |
Short DOI | https://doi.org/g8t3jb |
Share this

E-ISSN 2582-2160

CrossRef DOI is assigned to each research paper published in our journal.
IJFMR DOI prefix is
10.36948/ijfmr
Downloads
All research papers published on this website are licensed under Creative Commons Attribution-ShareAlike 4.0 International License, and all rights belong to their respective authors/researchers.
