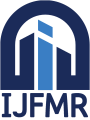
International Journal For Multidisciplinary Research
E-ISSN: 2582-2160
•
Impact Factor: 9.24
A Widely Indexed Open Access Peer Reviewed Multidisciplinary Bi-monthly Scholarly International Journal
Home
Research Paper
Submit Research Paper
Publication Guidelines
Publication Charges
Upload Documents
Track Status / Pay Fees / Download Publication Certi.
Editors & Reviewers
View All
Join as a Reviewer
Get Membership Certificate
Current Issue
Publication Archive
Conference
Publishing Conf. with IJFMR
Upcoming Conference(s) ↓
WSMCDD-2025
GSMCDD-2025
Conferences Published ↓
RBS:RH-COVID-19 (2023)
ICMRS'23
PIPRDA-2023
Contact Us
Plagiarism is checked by the leading plagiarism checker
Call for Paper
Volume 7 Issue 2
March-April 2025
Indexing Partners






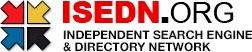

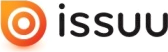


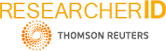
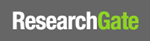
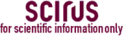
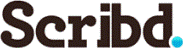

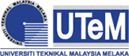
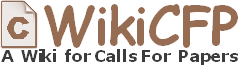
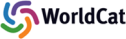
Dynamic Feature Engineering for Transparent Machine Learning: a Framework for Interpretable Model Explanations
Author(s) | Apurva Reddy Kistampally |
---|---|
Country | United States |
Abstract | This article introduces a comprehensive framework for dynamic feature engineering that enhances the transparency and interpretability of machine learning models across various industries. The framework addresses the critical challenge of making complex ML models more comprehensible to stakeholders while maintaining high-performance standards. Through a multi-layered architecture incorporating feature transformation, mapping methodologies, and modular templates, the system clearly explains model decisions to technical and non-technical users. The framework demonstrates significant improvements in model interpretability, stakeholder understanding, and operational efficiency across financial, healthcare, and customer engagement applications. Organizations can achieve enhanced model transparency without sacrificing accuracy by implementing structured feature mapping and automated optimization techniques. The article presents a detailed analysis of implementation strategies, performance metrics, and integration protocols, providing practitioners with actionable insights for deploying interpretable ML solutions. The article contributes to the growing field of explainable AI by offering a scalable, enterprise-ready framework that bridges the gap between technical complexity and business understanding. |
Keywords | Feature Engineering, Machine Learning Interpretability, Model Transparency, Explainable AI, Enterprise Framework |
Field | Computer |
Published In | Volume 6, Issue 6, November-December 2024 |
Published On | 2024-12-12 |
DOI | https://doi.org/10.36948/ijfmr.2024.v06i06.32651 |
Short DOI | https://doi.org/g8vgh4 |
Share this

E-ISSN 2582-2160

CrossRef DOI is assigned to each research paper published in our journal.
IJFMR DOI prefix is
10.36948/ijfmr
Downloads
All research papers published on this website are licensed under Creative Commons Attribution-ShareAlike 4.0 International License, and all rights belong to their respective authors/researchers.
