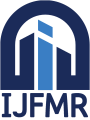
International Journal For Multidisciplinary Research
E-ISSN: 2582-2160
•
Impact Factor: 9.24
A Widely Indexed Open Access Peer Reviewed Multidisciplinary Bi-monthly Scholarly International Journal
Home
Research Paper
Submit Research Paper
Publication Guidelines
Publication Charges
Upload Documents
Track Status / Pay Fees / Download Publication Certi.
Editors & Reviewers
View All
Join as a Reviewer
Get Membership Certificate
Current Issue
Publication Archive
Conference
Publishing Conf. with IJFMR
Upcoming Conference(s) ↓
WSMCDD-2025
GSMCDD-2025
Conferences Published ↓
RBS:RH-COVID-19 (2023)
ICMRS'23
PIPRDA-2023
Contact Us
Plagiarism is checked by the leading plagiarism checker
Call for Paper
Volume 7 Issue 2
March-April 2025
Indexing Partners






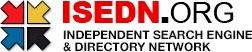

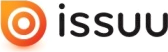


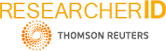
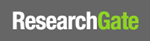
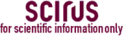
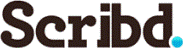

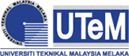
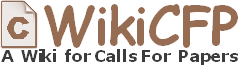
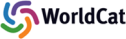
Advancements in Large Language Model Efficiency: A Literature Review on 1-bit Quantization
Author(s) | Lalitha Shree C P, Nethravathi B |
---|---|
Country | India |
Abstract | Large Language Models suffer from most of the challenges regarding computational cost, memory, and energy consumption, which makes their scaling difficult. BitNet b1.58 addresses these issues by introducing a novel 1.58-bit quantization with ternary weights {-1, 0, 1}. This can achieve performance comparable to FP16 models with much lower resource requirements. BitNet b1.58 provides 2.71x faster inference and 3.55x less memory usage than FP16 baselines. Its ternary weights allow for efficient feature filtering, making it a versatile choice for many AI applications. This makes it a standout solution, balancing high performance with resource efficiency. While BitNet b1.58 has its 1-bit mover heads that make it affordable for edge and mobile devices, it also allows longer sequences. This will mark further developments toward making AI scalable and resource-aware for various applications. |
Keywords | LLMs, 1 bit Quantization, BitNet, BitNet b1.58 |
Field | Engineering |
Published In | Volume 6, Issue 6, November-December 2024 |
Published On | 2024-12-10 |
DOI | https://doi.org/10.36948/ijfmr.2024.v06i06.32856 |
Short DOI | https://doi.org/g8vgf5 |
Share this

E-ISSN 2582-2160

CrossRef DOI is assigned to each research paper published in our journal.
IJFMR DOI prefix is
10.36948/ijfmr
Downloads
All research papers published on this website are licensed under Creative Commons Attribution-ShareAlike 4.0 International License, and all rights belong to their respective authors/researchers.
