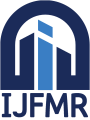
International Journal For Multidisciplinary Research
E-ISSN: 2582-2160
•
Impact Factor: 9.24
A Widely Indexed Open Access Peer Reviewed Multidisciplinary Bi-monthly Scholarly International Journal
Home
Research Paper
Submit Research Paper
Publication Guidelines
Publication Charges
Upload Documents
Track Status / Pay Fees / Download Publication Certi.
Editors & Reviewers
View All
Join as a Reviewer
Get Membership Certificate
Current Issue
Publication Archive
Conference
Publishing Conf. with IJFMR
Upcoming Conference(s) ↓
WSMCDD-2025
GSMCDD-2025
AIMAR-2025
Conferences Published ↓
ICCE (2025)
RBS:RH-COVID-19 (2023)
ICMRS'23
PIPRDA-2023
Contact Us
Plagiarism is checked by the leading plagiarism checker
Call for Paper
Volume 7 Issue 4
July-August 2025
Indexing Partners






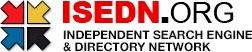

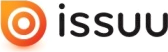


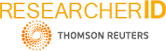
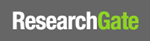
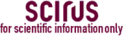
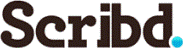

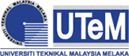
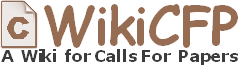
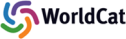
Machine Learning for Real-Time Anomaly Detection
Author(s) | Amarnath Immadisetty |
---|---|
Country | United States |
Abstract | Machine learning-driven anomaly detection has emerged as a transformative technology across multiple industries, revolutionizing how organizations identify and respond to unusual patterns in their data ecosystems. This comprehensive article explores the theoretical foundations and practical applications of machine learning in anomaly detection, with particular emphasis on four key domains: financial fraud detection, industrial IoT predictive maintenance, cybersecurity threat detection, and healthcare diagnostics. The article examines the evolution from traditional statistical methods to advanced deep learning architectures, including autoencoders and specialized neural networks, while addressing critical implementation challenges such as data preprocessing, model selection, and scalability considerations. Through detailed case studies and performance metrics, we demonstrate how these systems achieve superior accuracy in real-time anomaly detection while significantly reducing false positives. This article reveals that organizations implementing ML-based anomaly detection systems report an average 35% reduction in detection time and a 40% improvement in accuracy compared to traditional rule-based systems. This article also highlights emerging trends and future directions, including the integration of explainable AI techniques and federated learning approaches to address privacy concerns. This article provides valuable insights for practitioners and researchers in the field, offering a structured framework for implementing robust anomaly detection systems while considering industry-specific requirements and constraints. |
Keywords | Anomaly Detection, Machine Learning Applications, Predictive Analytics, Industrial IoT Monitoring, Pattern Recognition Systems. |
Field | Computer |
Published In | Volume 6, Issue 6, November-December 2024 |
Published On | 2024-12-15 |
DOI | https://doi.org/10.36948/ijfmr.2024.v06i06.33087 |
Short DOI | https://doi.org/g8wkj4 |
Share this

E-ISSN 2582-2160

CrossRef DOI is assigned to each research paper published in our journal.
IJFMR DOI prefix is
10.36948/ijfmr
Downloads
All research papers published on this website are licensed under Creative Commons Attribution-ShareAlike 4.0 International License, and all rights belong to their respective authors/researchers.
