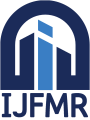
International Journal For Multidisciplinary Research
E-ISSN: 2582-2160
•
Impact Factor: 9.24
A Widely Indexed Open Access Peer Reviewed Multidisciplinary Bi-monthly Scholarly International Journal
Home
Research Paper
Submit Research Paper
Publication Guidelines
Publication Charges
Upload Documents
Track Status / Pay Fees / Download Publication Certi.
Editors & Reviewers
View All
Join as a Reviewer
Get Membership Certificate
Current Issue
Publication Archive
Conference
Publishing Conf. with IJFMR
Upcoming Conference(s) ↓
WSMCDD-2025
GSMCDD-2025
Conferences Published ↓
RBS:RH-COVID-19 (2023)
ICMRS'23
PIPRDA-2023
Contact Us
Plagiarism is checked by the leading plagiarism checker
Call for Paper
Volume 7 Issue 2
March-April 2025
Indexing Partners






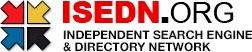

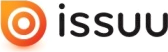


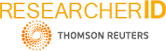
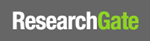
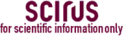
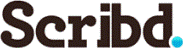

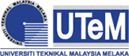
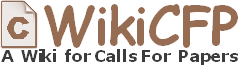
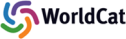
An Enhancement of AdaBoost Algorithm Applied In Online Transaction Fraud Detection System
Author(s) | Christian S. Ortega, Lance Daniel P. Lim, Alrhia Ruby S. Bautista, Vivien A. Agustin |
---|---|
Country | Philippines |
Abstract | This study is focused on the enhancement of the AdaBoost model for online transaction fraud detection to improve performance in detecting fraudulent activities. The study addresses the limitations of AdaBoost, including class imbalance, long training times, and overfitting. Three techniques were integrated to optimize the model. SMOTE (Synthetic Minority Over-sampling Technique) to balance the dataset, Quantile-Based Capping (QBC) to reduce training time, and Early Stopping to prevent overfitting. The dataset used for training consisted of online transaction records, with model performance evaluated using standard classification metrics. Results show that applying Heron-Centroid SMOTE led to an increase in accuracy, from 0.8150 to 0.8198, while significantly improving recall from 0.2962 to 0.3773, indicating better identification of minority class instances. The F-measure rose from 0.4135 to 0.4798, reflecting a better balance between precision and recall. The G-mean improved from 0.5337 to 0.5970, showing overall better classification performance. QBC reduced training time from 1.45 to 1.32 seconds, and Early Stopping increased accuracy from 0.73 to 0.816. These findings suggest that the proposed enhancements significantly improve AdaBoost’s efficiency and reliability, making it more effective for online fraud detection. |
Keywords | Adaboost Algorithm, Fraud Detection System, SMOTE, QBC, Early Stopping, Machine Learning |
Field | Computer |
Published In | Volume 6, Issue 6, November-December 2024 |
Published On | 2024-12-18 |
DOI | https://doi.org/10.36948/ijfmr.2024.v06i06.33132 |
Short DOI | https://doi.org/g8wkjh |
Share this

E-ISSN 2582-2160

CrossRef DOI is assigned to each research paper published in our journal.
IJFMR DOI prefix is
10.36948/ijfmr
Downloads
All research papers published on this website are licensed under Creative Commons Attribution-ShareAlike 4.0 International License, and all rights belong to their respective authors/researchers.
