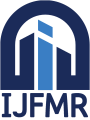
International Journal For Multidisciplinary Research
E-ISSN: 2582-2160
•
Impact Factor: 9.24
A Widely Indexed Open Access Peer Reviewed Multidisciplinary Bi-monthly Scholarly International Journal
Home
Research Paper
Submit Research Paper
Publication Guidelines
Publication Charges
Upload Documents
Track Status / Pay Fees / Download Publication Certi.
Editors & Reviewers
View All
Join as a Reviewer
Get Membership Certificate
Current Issue
Publication Archive
Conference
Publishing Conf. with IJFMR
Upcoming Conference(s) ↓
WSMCDD-2025
GSMCDD-2025
Conferences Published ↓
RBS:RH-COVID-19 (2023)
ICMRS'23
PIPRDA-2023
Contact Us
Plagiarism is checked by the leading plagiarism checker
Call for Paper
Volume 7 Issue 2
March-April 2025
Indexing Partners






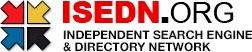

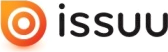


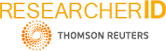
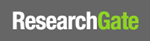
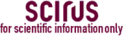
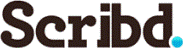

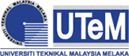
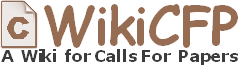
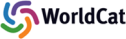
Comparative Analysis of Decision-Making Efficiency of Large Language Models
Author(s) | Mirza Niaz Zaman Elin |
---|---|
Country | Bangladesh |
Abstract | Large language models (LLMs) have emerged as powerful tools in the field of artificial intelligence (AI), attracting considerable attention from researchers and practitioners. These models demonstrate remarkable capabilities in various tasks, including decision-making. This paper aims to compare the decision-making efficiency of two prominent LLMs, Bard and GPT, across different domains.To conduct a comprehensive evaluation, a set of carefully designed questions was used to assess the performance of Bard and GPT in specific decision-making contexts. Through quantitative analysis, we aimed to quantify their abilities and identify potential variations in their performance.The results of our study revealed interesting insights into the decision-making efficiency of Bard and GPT across different domains. In the domain of logical reasoning and error detection, both Bard and GPT exhibited similar performance, but GPT outperformed Bard in data analysis by a notable margin. This finding suggests that GPT possesses stronger analytical abilities, enabling it to make more accurate and reliable decisions in contexts that require accurate data analysis and interpretation.The comparative analysis of Bard and GPT's decision-making efficiency highlights the significance of considering the specific domains and tasks when evaluating the performance of LLMs. It underscores the fact that different LLMs may possess domain-specific strengths and weaknesses, which can have a profound impact on their decision-making capabilities.Future research endeavors may involve expanding the evaluation to additional domains and considering a larger sample of questions to enhance the reliability and generalizability of the findings. Moreover, investigating the interpretability and explainability of LLMs in decision-making processes could shed further light on their decision-making strategies and enhance trust and transparency in their applications.This paper contributes to the growing body of research on LLMs by comparing the decision-making efficiency of Bard and GPT across different domains. The findings highlight the relative strengths of each model, emphasizing the importance of domain-specific considerations in decision-making tasks. By leveraging the capabilities of LLMs, practitioners can harness their potential to improve decision-making processes in diverse real-world applications. |
Keywords | large language models, decision-making, efficiency, Bard, GPT |
Field | Computer > Artificial Intelligence / Simulation / Virtual Reality |
Published In | Volume 5, Issue 3, May-June 2023 |
Published On | 2023-05-31 |
DOI | https://doi.org/10.36948/ijfmr.2023.v05i03.3342 |
Short DOI | https://doi.org/gr97s5 |
Share this

E-ISSN 2582-2160

CrossRef DOI is assigned to each research paper published in our journal.
IJFMR DOI prefix is
10.36948/ijfmr
Downloads
All research papers published on this website are licensed under Creative Commons Attribution-ShareAlike 4.0 International License, and all rights belong to their respective authors/researchers.
