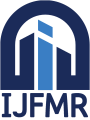
International Journal For Multidisciplinary Research
E-ISSN: 2582-2160
•
Impact Factor: 9.24
A Widely Indexed Open Access Peer Reviewed Multidisciplinary Bi-monthly Scholarly International Journal
Home
Research Paper
Submit Research Paper
Publication Guidelines
Publication Charges
Upload Documents
Track Status / Pay Fees / Download Publication Certi.
Editors & Reviewers
View All
Join as a Reviewer
Get Membership Certificate
Current Issue
Publication Archive
Conference
Publishing Conf. with IJFMR
Upcoming Conference(s) ↓
WSMCDD-2025
GSMCDD-2025
Conferences Published ↓
RBS:RH-COVID-19 (2023)
ICMRS'23
PIPRDA-2023
Contact Us
Plagiarism is checked by the leading plagiarism checker
Call for Paper
Volume 7 Issue 2
March-April 2025
Indexing Partners






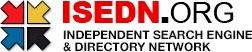

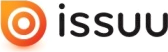


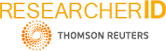
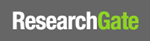
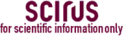
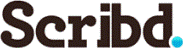

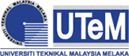
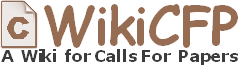
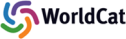
QWhale and SARSAWhale: Energy-Efficient and Energy-Aware Algorithms for High-Load Cloud Environments
Author(s) | Aakarshit Srivastava, Bhaskar Banerjee, Ayush Verma |
---|---|
Country | India |
Abstract | Cloud computing has transformed resource management by providing on-demand access to shared computational resources, yet task scheduling in dynamic environments remains a critical challenge due to fluctuating workloads, varying resource availability, and diverse task priorities. Traditional optimization algorithms often fail to adapt effectively to these dynamic conditions. This paper introduces two novel hybrid frameworks: the Q-Whale Algorithm (QWA) and the SARSA-Whale Algorithm (SWA). Both approaches integrate the Whale Optimization Algorithm (WOA) with reinforcement learning techniques—Q-learning and SARSA, respectively—to address the challenges of real-time task scheduling. QWA combines the global search and exploration capabilities of WOA with the adaptive decision-making of Q-learning, while SWA leverages SARSA’s on-policy learning mechanism for enhanced convergence and decision-making in dynamic settings. Experimental evaluations in simulated cloud environments reveal that both algorithms outperform traditional scheduling methods, with SWA demonstrating marginally better performance in terms of resource utilization, makespan reduction, and adherence to task deadlines. These findings highlight the potential of hybrid intelligent algorithms in advancing the efficiency and reliability of cloud-based task scheduling systems. |
Keywords | ReinforcementLearning,Qlearning,Whale,WOA,QWhale,SARSA,SARSAWhale |
Field | Computer > Artificial Intelligence / Simulation / Virtual Reality |
Published In | Volume 7, Issue 1, January-February 2025 |
Published On | 2025-01-10 |
DOI | https://doi.org/10.36948/ijfmr.2025.v07i01.34840 |
Short DOI | https://doi.org/g82gwq |
Share this

E-ISSN 2582-2160

CrossRef DOI is assigned to each research paper published in our journal.
IJFMR DOI prefix is
10.36948/ijfmr
Downloads
All research papers published on this website are licensed under Creative Commons Attribution-ShareAlike 4.0 International License, and all rights belong to their respective authors/researchers.
