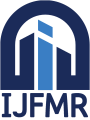
International Journal For Multidisciplinary Research
E-ISSN: 2582-2160
•
Impact Factor: 9.24
A Widely Indexed Open Access Peer Reviewed Multidisciplinary Bi-monthly Scholarly International Journal
Home
Research Paper
Submit Research Paper
Publication Guidelines
Publication Charges
Upload Documents
Track Status / Pay Fees / Download Publication Certi.
Editors & Reviewers
View All
Join as a Reviewer
Get Membership Certificate
Current Issue
Publication Archive
Conference
Publishing Conf. with IJFMR
Upcoming Conference(s) ↓
WSMCDD-2025
GSMCDD-2025
Conferences Published ↓
RBS:RH-COVID-19 (2023)
ICMRS'23
PIPRDA-2023
Contact Us
Plagiarism is checked by the leading plagiarism checker
Call for Paper
Volume 7 Issue 2
March-April 2025
Indexing Partners






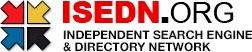

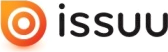


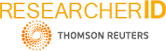
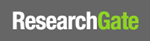
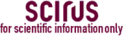
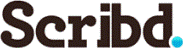

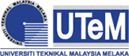
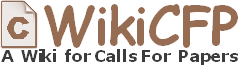
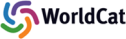
Deep Fake Video Detection
Author(s) | K. B. Nikumbh, Vaibhav Adhe, Sahil Karamkar, Shivam Ghaware, Shruti Khaire |
---|---|
Country | India |
Abstract | The growing computation power has made the deep learning algorithms so powerful that creating a indistinguishable human synthesized video popularly called as deep fakes have became very simple. Scenarios where these realistic face swapped deep fakes are used to create political distress, fake terrorism events, revenge porn, blackmail peoples are easily envisioned. In this work, we describe a new deep learning-based method that can effectively distinguish AI-generated fake videos from real videos.Our method is capable of automatically detecting the replacement and reenactment deep fakes. We are trying to use Artificial Intelligence(AI) to fight Artificial Intelligence(AI). Our system uses a Res-Next Convolution neural network to extract the frame-level features and these features and further used to train the Long Short Term Memory(LSTM) based Recurrent Neural Network(RNN) to classify whether the video is subject to any kind of manipulation or not, i.e whether the video is deep fake or real video. To emulate the real time scenarios and make the model perform better on real time data, we evaluate our method on large amount of balanced and mixed data-set prepared by mixing the various available data-set like Face-Forensic++[1], Deepfake detection challenge[2], and Celeb-DF[3]. We also show how our system can achieve competitive result using very simple and robust approach |
Keywords | Res-Next Convolution neural network. Recurrent Neural Network (RNN). Long Short Term Memory(LSTM). Computer vision |
Field | Engineering |
Published In | Volume 7, Issue 1, January-February 2025 |
Published On | 2025-01-23 |
DOI | https://doi.org/10.36948/ijfmr.2025.v07i01.35345 |
Short DOI | https://doi.org/g82wpw |
Share this

E-ISSN 2582-2160

CrossRef DOI is assigned to each research paper published in our journal.
IJFMR DOI prefix is
10.36948/ijfmr
Downloads
All research papers published on this website are licensed under Creative Commons Attribution-ShareAlike 4.0 International License, and all rights belong to their respective authors/researchers.
