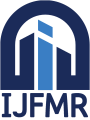
International Journal For Multidisciplinary Research
E-ISSN: 2582-2160
•
Impact Factor: 9.24
A Widely Indexed Open Access Peer Reviewed Multidisciplinary Bi-monthly Scholarly International Journal
Home
Research Paper
Submit Research Paper
Publication Guidelines
Publication Charges
Upload Documents
Track Status / Pay Fees / Download Publication Certi.
Editors & Reviewers
View All
Join as a Reviewer
Get Membership Certificate
Current Issue
Publication Archive
Conference
Publishing Conf. with IJFMR
Upcoming Conference(s) ↓
WSMCDD-2025
GSMCDD-2025
Conferences Published ↓
RBS:RH-COVID-19 (2023)
ICMRS'23
PIPRDA-2023
Contact Us
Plagiarism is checked by the leading plagiarism checker
Call for Paper
Volume 7 Issue 2
March-April 2025
Indexing Partners






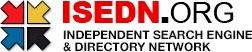

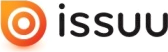


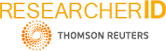
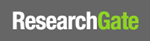
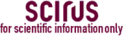
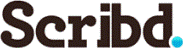

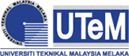
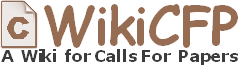
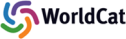
An Enhanced Social Media Android Malware Detection through Stacking Ensemble Machine Learning Model with Reinforcement Learning-Based Rule Factor
Author(s) | Saqib Malik, Narendra Sharma |
---|---|
Country | India |
Abstract | Android malware detection in social media dataset faces challenges such as feature selection, class imbalance, and efficient model construction. Imbalanced datasets reduce detection accuracy, necessitating strategies to balance data and enhance detection precision. This study aims to develop an ensemble learning-based model that tackles class imbalance, and ensures accurate and efficient malware detection. It also introduces a novel classifier for Android malware detection system that leverages reinforcement learning-based rules. Two sampling methods—random undersampling, and random oversampling—are applied to balance imbalanced datasets. The proposed Stacking Model with Reinforcement Learning-Based Rule Factor (SMRLF) combines classifiers such as stochastic gradient tree boosting (SGTB), Decision Tree (DT), Logistic Regression (LR) and Naïve Bias (NB) for android malware detection and employs reinforcement learning-based rules to enhance classification precision and validation. The SMRLF model achieves 99.00% detection accuracy, outperforming alternative classifiers like LR (95.00%), Naïve Bayes (67.50%), DT (93.20%), and meta-learner (98.40%). It also demonstrates reduced computational time (24.872 seconds) compared to others. The study introduces a novel SMRLF classifier that combines reinforcement learning-based rules factor with ensemble learning techniques, offering a robust solution for Android malware detection with high accuracy and efficiency. This work represents a major breakthrough in stacking techniques by presenting a novel combination of classifiers intended to improve accuracy. This study highlight the value of ensemble approaches, especially stacking, in enhancing Android malware detection rates by assessing classifier performances using a new association of two social media datasets. In addition to improving detection accuracy, this method offers a framework that may be used for various classification problems. Beyond malware detection, the knowledge gathered from this effort provides insightful viewpoints for more general categorization applications. Sustained efficacy in detection systems depends on continued research into developing malware approaches in order to preserve and grow these achievements. |
Keywords | Android malware detection, social media, ensemble learning, Stacking Model, Reinforcement Learning-Based Rule Factor. |
Field | Computer Applications |
Published In | Volume 7, Issue 1, January-February 2025 |
Published On | 2025-02-01 |
DOI | https://doi.org/10.36948/ijfmr.2025.v07i01.36177 |
Short DOI | https://doi.org/g834cs |
Share this

E-ISSN 2582-2160

CrossRef DOI is assigned to each research paper published in our journal.
IJFMR DOI prefix is
10.36948/ijfmr
Downloads
All research papers published on this website are licensed under Creative Commons Attribution-ShareAlike 4.0 International License, and all rights belong to their respective authors/researchers.
