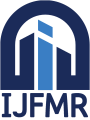
International Journal For Multidisciplinary Research
E-ISSN: 2582-2160
•
Impact Factor: 9.24
A Widely Indexed Open Access Peer Reviewed Multidisciplinary Bi-monthly Scholarly International Journal
Home
Research Paper
Submit Research Paper
Publication Guidelines
Publication Charges
Upload Documents
Track Status / Pay Fees / Download Publication Certi.
Editors & Reviewers
View All
Join as a Reviewer
Get Membership Certificate
Current Issue
Publication Archive
Conference
Publishing Conf. with IJFMR
Upcoming Conference(s) ↓
WSMCDD-2025
GSMCDD-2025
Conferences Published ↓
RBS:RH-COVID-19 (2023)
ICMRS'23
PIPRDA-2023
Contact Us
Plagiarism is checked by the leading plagiarism checker
Call for Paper
Volume 7 Issue 2
March-April 2025
Indexing Partners






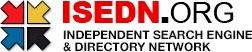

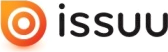


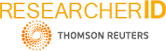
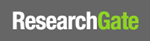
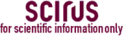
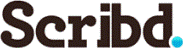

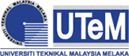
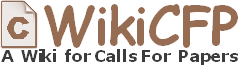
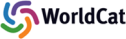
Combined hybrid feature selection and classification for heart disease prediction in the cloud-based IoT health care system using Machine Learning
Author(s) | N.Keerthika, Dr.S.Nithyanandam |
---|---|
Country | India |
Abstract | Health care Management System (HMS) is a key to successful management of any health care industry. Health care management system has so many research dimensions such as identifying disease and diagnostic, drug discovery manufacturing, Bioinformatics’ problem, personalized treatments, Patient image analysis and so on. Heart Disease Prediction (HDP) is a process of identifying heart disease in advance and recognizes patient health condition by applying techniques on patient heart related symptoms. Now a day’s the problem of identifying heart diseases are solved by machine learning techniques. In this paper we are constructed heart disease prediction method using combined feature selection and classification machine learning techniques. According to the existing study the one of the main difficult in heart disease prediction system is that the available data in open sources are not properly recorded the necessary characteristics and also there is some lagging in finding the useful features from the available features. The process of removing inappropriate features from an available feature set while preserving sufficient classification accuracy is known as feature selection. A methodology is proposed in this paper that consists of two phases: Phase one employs two broad categories of feature selection techniques to identify the efficient feature sets and it is given to the input of our second phase such as classification. In this work we will concentrated on filter based method for feature selection such as Chi-square, Fast Correlation Based Filter (FCBF), Gini Index (GI), RelifeF, and wrapper based method for feature selection such as Backward Feature Elimination (BFE), Exhaustive Feature Selection (EFS), Forward Feature Selection (FFS), and Recursive Feature Elimination (RFE). The UCI heart disease data set is used to evaluate the output in this study. Finally, the proposed system's performance is validated by various experiments setups. |
Keywords | Health care Management System, Heart Disease Prediction, machine learning techniques, feature selection techniques, classification, Filter FS, Wrapper FS, FCBF, EFS, FFS, RFE, BFE, Chi-square, GI, RelifeF. |
Field | Engineering |
Published In | Volume 7, Issue 1, January-February 2025 |
Published On | 2025-02-02 |
DOI | https://doi.org/10.36948/ijfmr.2025.v07i01.36269 |
Short DOI | https://doi.org/g83xw6 |
Share this

E-ISSN 2582-2160

CrossRef DOI is assigned to each research paper published in our journal.
IJFMR DOI prefix is
10.36948/ijfmr
Downloads
All research papers published on this website are licensed under Creative Commons Attribution-ShareAlike 4.0 International License, and all rights belong to their respective authors/researchers.
