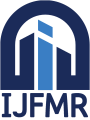
International Journal For Multidisciplinary Research
E-ISSN: 2582-2160
•
Impact Factor: 9.24
A Widely Indexed Open Access Peer Reviewed Multidisciplinary Bi-monthly Scholarly International Journal
Home
Research Paper
Submit Research Paper
Publication Guidelines
Publication Charges
Upload Documents
Track Status / Pay Fees / Download Publication Certi.
Editors & Reviewers
View All
Join as a Reviewer
Get Membership Certificate
Current Issue
Publication Archive
Conference
Publishing Conf. with IJFMR
Upcoming Conference(s) ↓
WSMCDD-2025
GSMCDD-2025
AIMAR-2025
Conferences Published ↓
ICCE (2025)
RBS:RH-COVID-19 (2023)
ICMRS'23
PIPRDA-2023
Contact Us
Plagiarism is checked by the leading plagiarism checker
Call for Paper
Volume 7 Issue 4
July-August 2025
Indexing Partners






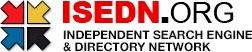

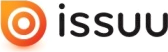


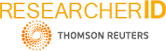
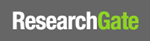
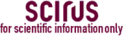
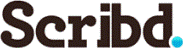

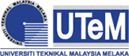
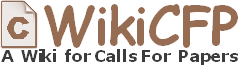
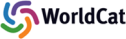
A Cascading Ensemble with Custom Subset Generation and Multi-level Fusion for Enhanced Breast Cancer Detection
Author(s) | Vandana Lingampally, K.Radhika |
---|---|
Country | India |
Abstract | The ensemble learning technique is a powerful method that combines multiple machine learning models to address their individual limitations and create an optimized predictive model. By harnessing the strengths of each model, ensemble learning significantly enhances overall performance, making it particularly valuable for classification tasks across diverse domains. In this study, an efficient three-level stacking ensemble approach is proposed for diagnosing breast cancer. At the first level, a diverse set of base learners is employed, encompassing decision trees, logistic regression, k-nearest neighbors, support vector machines, and Gaussian naive Bayes. Each first-level learner is trained on distinct subsets of the training data with 10-fold cross-validation, thereby ensuring model robustness and mitigating the risk of overfitting. Transitioning to the second level, sophisticated ensemble models including Adaboost, GBM, and Random forest are introduced. These second-level learners undergo training using the validation predictions generated by the first-level models, while incorporating the top six informative features extracted from the dataset. To enhance their performance and mitigate overfitting, the models are optimized through the application of 10-fold cross-validation. In order to achieve further refinement of the ensemble, a third-level learner, represented by a deep neural network (DNN), is introduced. This DNN is trained on the validation predictions obtained from both the first and second levels, facilitating the capturing and synthesis of the collective knowledge of the ensemble. By leveraging the inherent capabilities of deep learning, the DNN maximizes the amalgamated benefits derived from the preceding levels, resulting in a substantial enhancement of the overall predictive power. The effectiveness of the ensemble approach is evaluated using well-established performance metrics, including accuracy, sensitivity, and specificity, which are derived from the analysis of the confusion matrix. The results incontrovertibly demonstrate that the ensemble models surpass individual machine learning models in accurately detecting breast cancer. |
Keywords | breast cancer, machine learning, ensemble techniques, stacking |
Field | Engineering |
Published In | Volume 5, Issue 3, May-June 2023 |
Published On | 2023-06-13 |
DOI | https://doi.org/10.36948/ijfmr.2023.v05i03.3662 |
Short DOI | https://doi.org/gscdpt |
Share this

E-ISSN 2582-2160

CrossRef DOI is assigned to each research paper published in our journal.
IJFMR DOI prefix is
10.36948/ijfmr
Downloads
All research papers published on this website are licensed under Creative Commons Attribution-ShareAlike 4.0 International License, and all rights belong to their respective authors/researchers.
