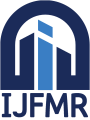
International Journal For Multidisciplinary Research
E-ISSN: 2582-2160
•
Impact Factor: 9.24
A Widely Indexed Open Access Peer Reviewed Multidisciplinary Bi-monthly Scholarly International Journal
Home
Research Paper
Submit Research Paper
Publication Guidelines
Publication Charges
Upload Documents
Track Status / Pay Fees / Download Publication Certi.
Editors & Reviewers
View All
Join as a Reviewer
Get Membership Certificate
Current Issue
Publication Archive
Conference
Publishing Conf. with IJFMR
Upcoming Conference(s) ↓
WSMCDD-2025
GSMCDD-2025
Conferences Published ↓
RBS:RH-COVID-19 (2023)
ICMRS'23
PIPRDA-2023
Contact Us
Plagiarism is checked by the leading plagiarism checker
Call for Paper
Volume 7 Issue 2
March-April 2025
Indexing Partners






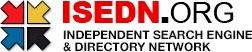

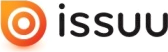


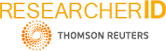
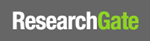
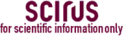
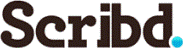

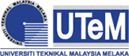
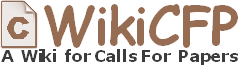
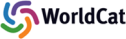
Generative Adversarial Networks for Historical Data Generation in Semiconductor Manufacturing: Applications and Challenges
Author(s) | Tarun Parmar |
---|---|
Country | United States |
Abstract | Semiconductor manufacturing relies heavily on historical data for process optimization, predictive modeling, and digital twin creation. However, obtaining comprehensive historical datasets can be challenging because of various factors such as equipment upgrades, process changes, and limited data collection. Generative Adversarial Networks (GANs) have emerged as a promising solution to this issue by generating synthetic data that closely resemble real-world distributions. This study explored the application of GANs to generate historical data for semiconductor fabrication facilities. We discuss the importance of data quality, the challenges in modeling complex fab operations, and the need to maintain data privacy. We also examined specialized GAN architectures suited for time-series data generation, multimodal GANs for handling different data types, and hierarchical GANs for capturing relationships between equipment, processes, and fab-wide metrics. Training approaches, evaluation metrics, and potential applications of GAN-generated data in fab simulations, predictive maintenance, and process optimization are also discussed. Furthermore, we address concerns regarding the reliability of the synthetic data, potential biases, and computational requirements. Finally, we highlight future research directions, including the integration of GANs with other AI technologies and the possibility of creating digital twins in entire fab facilities. While challenges remain, GANs present a promising avenue for enhancing semiconductor manufacturing through improved predictive modeling and digital twin creation. |
Keywords | semiconductor manufacturing, Generative Adversarial Networks (GANs), historical data, synthetic data generation, predictive modeling, digital twins, hierarchical models, fab simulations |
Field | Engineering |
Published In | Volume 6, Issue 6, November-December 2024 |
Published On | 2024-12-04 |
DOI | https://doi.org/10.36948/ijfmr.2024.v06i06.37230 |
Short DOI | https://doi.org/ |
Share this

E-ISSN 2582-2160

CrossRef DOI is assigned to each research paper published in our journal.
IJFMR DOI prefix is
10.36948/ijfmr
Downloads
All research papers published on this website are licensed under Creative Commons Attribution-ShareAlike 4.0 International License, and all rights belong to their respective authors/researchers.
