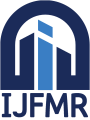
International Journal For Multidisciplinary Research
E-ISSN: 2582-2160
•
Impact Factor: 9.24
A Widely Indexed Open Access Peer Reviewed Multidisciplinary Bi-monthly Scholarly International Journal
Home
Research Paper
Submit Research Paper
Publication Guidelines
Publication Charges
Upload Documents
Track Status / Pay Fees / Download Publication Certi.
Editors & Reviewers
View All
Join as a Reviewer
Get Membership Certificate
Current Issue
Publication Archive
Conference
Publishing Conf. with IJFMR
Upcoming Conference(s) ↓
WSMCDD-2025
GSMCDD-2025
Conferences Published ↓
RBS:RH-COVID-19 (2023)
ICMRS'23
PIPRDA-2023
Contact Us
Plagiarism is checked by the leading plagiarism checker
Call for Paper
Volume 7 Issue 2
March-April 2025
Indexing Partners






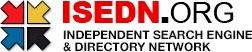

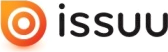


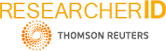
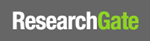
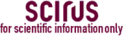
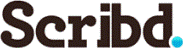

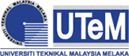
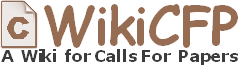
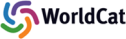
Query Optimization in Elasticsearch: A Comparative Analysis of Ranking Strategies
Author(s) | Ritesh Kumar |
---|---|
Country | United States |
Abstract | Elasticsearch is a widely used distributed search engine, powering applications in enterprise search, e-commerce, security analytics, and knowledge retrieval. As datasets grow, ensuring efficient query execution, accurate ranking, and system scalability becomes a critical challenge. This paper presents a comparative analysis of ranking strategies within Elasticsearch, including BM25 (default model), TF-IDF, Function Score Queries, Learning-to-Rank (LTR), and Vector Search. We evaluate their impact on query performance, retrieval accuracy, and computational efficiency. Additionally, we explore index-level and query-level optimizations, providing practical recommendations for search efficiency. Through real-world case studies, we demonstrate how different ranking models enhance enterprise search, security analytics, and personalized search applications. Finally, we discuss emerging trends such as AI-driven search, hybrid models, and neural ranking techniques, outlining future directions in Elasticsearch ranking optimization. Our findings suggest that while BM25 remains a strong baseline, hybrid models that integrate Function Score Queries, LTR, and Vector Search achieve an optimal balance among precision, recall, and scalability in large-scale applications. |
Keywords | Elasticsearch, Query Optimization, Ranking Strategies, BM25, Learning-to-Rank (LTR), Function Score Queries, Vector Search, Neural Ranking, Search Performance, Semantic Search, Information Retrieval, Search Relevance |
Field | Engineering |
Published In | Volume 6, Issue 1, January-February 2024 |
Published On | 2024-02-06 |
DOI | https://doi.org/10.36948/ijfmr.2024.v06i01.38173 |
Short DOI | https://doi.org/g86xsj |
Share this

E-ISSN 2582-2160

CrossRef DOI is assigned to each research paper published in our journal.
IJFMR DOI prefix is
10.36948/ijfmr
Downloads
All research papers published on this website are licensed under Creative Commons Attribution-ShareAlike 4.0 International License, and all rights belong to their respective authors/researchers.
