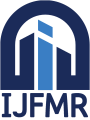
International Journal For Multidisciplinary Research
E-ISSN: 2582-2160
•
Impact Factor: 9.24
A Widely Indexed Open Access Peer Reviewed Multidisciplinary Bi-monthly Scholarly International Journal
Home
Research Paper
Submit Research Paper
Publication Guidelines
Publication Charges
Upload Documents
Track Status / Pay Fees / Download Publication Certi.
Editors & Reviewers
View All
Join as a Reviewer
Get Membership Certificate
Current Issue
Publication Archive
Conference
Publishing Conf. with IJFMR
Upcoming Conference(s) ↓
WSMCDD-2025
GSMCDD-2025
Conferences Published ↓
RBS:RH-COVID-19 (2023)
ICMRS'23
PIPRDA-2023
Contact Us
Plagiarism is checked by the leading plagiarism checker
Call for Paper
Volume 7 Issue 2
March-April 2025
Indexing Partners






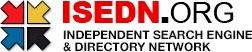

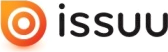


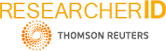
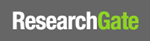
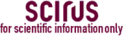
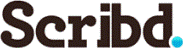

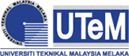
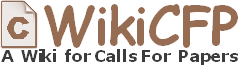
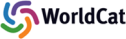
Comparative Analysis Of Data Lakes And Data Warehouses For Machine Learning
Author(s) | Bhanu Prakash Reddy Rella |
---|---|
Country | United States |
Abstract | The selection of the best data storage and management system stands essential because machine learning (ML) continues expanding its industry-driven innovation. Today's two most prevalent large-scale data processing systems are data lakes and data warehouses, which provide unique strengths and barriers when applied to ML workloads. This paper thoroughly compares data lakes and data warehouses by Analyzing their operational speed, abilities, and price efficiency alongside data, management controls, and ML integration capabilities. Data lakes showcase their superiority in processing unstructured together with semi-structured information because they serve deep learning and big data analytics requirements. A data warehouse offers optimized querying and structured storage, which is suitable for traditional business intelligence applications and ML platforms. The execution speed of data warehouses is faster compared to data lakes, but data lakes enable enhanced real-time abilities and flexibility for large-scale ML work. The research approach consists of conducting a feature-based examination of both architectures, combined with real-world examples, performance scaling data, and cost measurements. This research finds that AI analytics operate most successfully through data lakes; however, structured ML jobs need data warehouses for efficient operation. The combination of data lakehouse technology presents a new possibility for joining both paradigms to create more efficient environments for machine learning applications. |
Keywords | Data Lakes, Data Warehouses, Machine Learning Workloads, Big Data Analytics, Data Storage Architectures, Lakehouse Architecture, AI-optimized databases. |
Published In | Volume 7, Issue 2, March-April 2025 |
Published On | 2025-03-13 |
DOI | https://doi.org/10.36948/ijfmr.2025.v07i02.38869 |
Short DOI | https://doi.org/g8938x |
Share this

E-ISSN 2582-2160

CrossRef DOI is assigned to each research paper published in our journal.
IJFMR DOI prefix is
10.36948/ijfmr
Downloads
All research papers published on this website are licensed under Creative Commons Attribution-ShareAlike 4.0 International License, and all rights belong to their respective authors/researchers.
