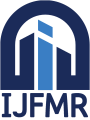
International Journal For Multidisciplinary Research
E-ISSN: 2582-2160
•
Impact Factor: 9.24
A Widely Indexed Open Access Peer Reviewed Multidisciplinary Bi-monthly Scholarly International Journal
Home
Research Paper
Submit Research Paper
Publication Guidelines
Publication Charges
Upload Documents
Track Status / Pay Fees / Download Publication Certi.
Editors & Reviewers
View All
Join as a Reviewer
Get Membership Certificate
Current Issue
Publication Archive
Conference
Publishing Conf. with IJFMR
Upcoming Conference(s) ↓
WSMCDD-2025
GSMCDD-2025
Conferences Published ↓
RBS:RH-COVID-19 (2023)
ICMRS'23
PIPRDA-2023
Contact Us
Plagiarism is checked by the leading plagiarism checker
Call for Paper
Volume 7 Issue 2
March-April 2025
Indexing Partners






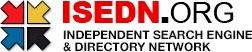

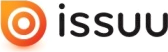


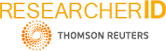
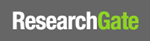
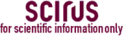
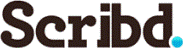

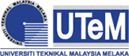
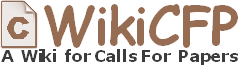
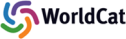
A Hybrid Approach for Leaf Disease DetectionUsing Convolutional Neural Networks and VisionTransformers
Author(s) | Ayush Sharma, Ayush Singh, Atul Kumar, Ayushi Tyagi, satendra Kumar |
---|---|
Country | India |
Abstract | Leaf diseases are a major problem affecting crop yield and food security in agriculture. Earlyand accurate detection is essential for timely intervention; however, traditional investigationmethods are often labor-intensive, time-consuming, and error-prone. This study presents ahybrid model combining convolutional neural networks (CNN) and visual transformation(ViT) to overcome these problems and improve the accuracy of disease detection. The modeluses CNN to capture local features such as edges and texture, while ViT to capture globalrelationships and generate powerful and effective solutions for disease detection. Use PlantVillage information. The data were previously processed, including data enhancementtechniques such as resizing, normalization and translation, and brightness adjustment toimprove the model.This hybrid model outperformed the CNN and ViT model by achieving 95% accuracy forAlternaria leaf spot and 97.38% accuracy for disease. More importantly, the model uses only9,913 training errors, reducing the computational load and training time withoutcompromising the accuracy and reliability expectations. The proposed model providesefficient and computationally efficient solutions for plant disease detection by combiningCNN and ViT. Its low requirements make it suitable for use in a real agriculturalenvironment, especially in limited areas.Additionally, this approach helps mitigate the misuse of chemical treatments by enablingprecise disease identification, contributing to sustainable agricultural practices.Future research will explore expanding the dataset to include additional disease classes,optimizing the model for edge devices to support real-time diagnostics, and integrating thesystem into mobile applications to empower farmers globally. This work provides afoundation for modernizing agricultural disease management through advanced machinelearning techniques. |
Keywords | Machine literacy, Alternaria Leaf Spot, Convolutional Neural Network, Vision Transformer, Leaf Disease Detection, and Plant Health |
Field | Computer > Artificial Intelligence / Simulation / Virtual Reality |
Published In | Volume 7, Issue 2, March-April 2025 |
Published On | 2025-03-24 |
DOI | https://doi.org/10.36948/ijfmr.2025.v07i02.38929 |
Short DOI | https://doi.org/g8937h |
Share this

E-ISSN 2582-2160

CrossRef DOI is assigned to each research paper published in our journal.
IJFMR DOI prefix is
10.36948/ijfmr
Downloads
All research papers published on this website are licensed under Creative Commons Attribution-ShareAlike 4.0 International License, and all rights belong to their respective authors/researchers.
