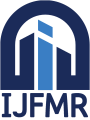
International Journal For Multidisciplinary Research
E-ISSN: 2582-2160
•
Impact Factor: 9.24
A Widely Indexed Open Access Peer Reviewed Multidisciplinary Bi-monthly Scholarly International Journal
Home
Research Paper
Submit Research Paper
Publication Guidelines
Publication Charges
Upload Documents
Track Status / Pay Fees / Download Publication Certi.
Editors & Reviewers
View All
Join as a Reviewer
Get Membership Certificate
Current Issue
Publication Archive
Conference
Publishing Conf. with IJFMR
Upcoming Conference(s) ↓
WSMCDD-2025
GSMCDD-2025
Conferences Published ↓
RBS:RH-COVID-19 (2023)
ICMRS'23
PIPRDA-2023
Contact Us
Plagiarism is checked by the leading plagiarism checker
Call for Paper
Volume 7 Issue 2
March-April 2025
Indexing Partners






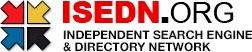

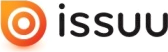


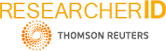
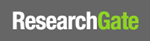
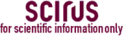
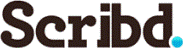

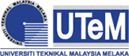
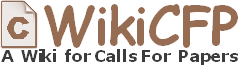
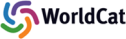
Enhancing Botnet Detection With Machine Learning And Explainable AI: A Step Towards Trustworthy AI Security
Author(s) | Mr. Vishva Patel, Hitasvi Shukla, Aashka Raval |
---|---|
Country | India |
Abstract | The rapid proliferation of botnets, armies of compromised machines controlled by malicious actors remotely, has played a pivotal role in the increase in cyber-attacks, such as Distributed Denial-of-Service (DDoS) attacks, credential theft, data exfiltration, command-and-control (C2) activity, and automated exploitation of vulnerabilities. Legacy botnet detection methods, founded on signature matching and deep packet inspection (DPI), are rapidly becoming a relic of the past because of the prevalence of encryption schemes like TLS 1.3, DNS-over-HTTPS (DoH), and encrypted VPN tunneling. These encryption mechanisms conceal packet payloads, making traditional network monitoring technology unsuitable for botnet detection. Faced with the challenge, ML-based botnet detection mechanisms have risen to the top. Existing ML-based approaches, however, are marred by two inherent weaknesses: (1) Lack of granularity in detection because most models are based on binary classification, with no distinction of botnet attack variants, and (2) Uninterpretability, where high-performing AI models behave like black-box mechanisms, which limits trust in security automation and leads to high false positives, thereby making threat analysis difficult for security practitioners. To overcome these challenges, this study proposes an AI-based, multi-class classification botnet detection system for encrypted network traffic that includes Explainable AI (XAI) techniques for improving model explainability and decision transparency. Two datasets, CICIDS-2017 and CTU-NCC, are used in this study, where a systematic data preprocessing step was employed to maximise data quality, feature representation, and model performance. Preprocessing included duplicate record removal, missing and infinite value imputation, categorical feature transformation, and removal of highly correlated and zero-variance features to minimise model bias. Dimensionality reduction was performed using Principal Component Analysis (PCA), lowering features of CICIDS-2017 from 70 to 34 and those of CTU-NCC from 17 to 4 for maximizing computational efficiency. Additionally, to deal with skewed class distributions, Synthetic Minority Over-Sampling Technique (SMOTE) was employed to synthesise minority class samples to offer balanced representation of botnet attack types. For CICIDS-2017, we used three machine learning algorithms: Random Forest (RF) with cross-validation (0.98 accuracy, 100K samples per class), eXtreme Gradient Boosting (XGB) with Bayesian optimisation (0.997 accuracy, 180K samples per class), and our recently introduced Hybrid K-Nearest Neighbours(KNN) + Random Forest (RF) model, resulting in state-of-the-art accuracy of 0.99 (180K samples per class). The CTU-NCC dataset was divided across three network sensors and processed separately. Random Forest (RF), Decision Tree (DT), and KNN models were trained independently for each sensor, and to enhance performance, ensemble learning methods such as stacking and voting were applied to combine the results from each of the sensors. The resulting accuracies were as follows: (Random Forest Stacking: 99.38%, Random Forest Voting: 99.35% ), (Decision Tree Stacking: 99.68%, Decision Tree Voting: 91.65%), and (KNN Stacking: 97.53%, KNN Voting: 97.11%). Explainable AI (XAI) techniques like SHAP (SHapley Additive exPlanations) and LIME (Local Interpretable Model agnostic Explanation) were integrated to provide enhanced interpretability in eXtreme Gradient Boosting and our Hybrid KNN+Random Forest model, which provided explanations for model decisions and enhanced analyst confidence in the system prediction. Our key contribution is the Hybrid KNN+Random Forest system with 0.99 accuracy and provision of explainability. We illustrate an accurate, scalable, and deployable AI-based solution for botnet attacks. Our experimentation shows that the multi-class classification method greatly assists in botnet attack discrimination, and Explainable AI (XAI) helps enhance clarity and is thus a strong, practical solution in the real case of botnet detection in an encrypted network scenario. |
Keywords | Botnet Detection, Encrypted Networks, Ensemble Models, Explainable AI |
Field | Computer > Network / Security |
Published In | Volume 7, Issue 2, March-April 2025 |
Published On | 2025-03-17 |
DOI | https://doi.org/10.36948/ijfmr.2025.v07i02.39353 |
Short DOI | https://doi.org/g882hr |
Share this

E-ISSN 2582-2160

CrossRef DOI is assigned to each research paper published in our journal.
IJFMR DOI prefix is
10.36948/ijfmr
Downloads
All research papers published on this website are licensed under Creative Commons Attribution-ShareAlike 4.0 International License, and all rights belong to their respective authors/researchers.
