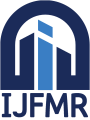
International Journal For Multidisciplinary Research
E-ISSN: 2582-2160
•
Impact Factor: 9.24
A Widely Indexed Open Access Peer Reviewed Multidisciplinary Bi-monthly Scholarly International Journal
Home
Research Paper
Submit Research Paper
Publication Guidelines
Publication Charges
Upload Documents
Track Status / Pay Fees / Download Publication Certi.
Editors & Reviewers
View All
Join as a Reviewer
Get Membership Certificate
Current Issue
Publication Archive
Conference
Publishing Conf. with IJFMR
Upcoming Conference(s) ↓
WSMCDD-2025
GSMCDD-2025
Conferences Published ↓
RBS:RH-COVID-19 (2023)
ICMRS'23
PIPRDA-2023
Contact Us
Plagiarism is checked by the leading plagiarism checker
Call for Paper
Volume 7 Issue 2
March-April 2025
Indexing Partners






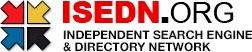

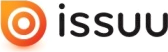


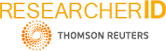
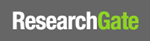
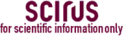
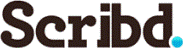

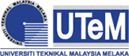
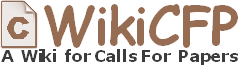
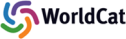
Optimizing Cloud Costs Through AI-Driven Workload Distribution
Author(s) | Mr. Greesham Anand, Prasanna Sankaran, Sambhav Patil |
---|---|
Country | India |
Abstract | This research explores the optimization of cloud computing costs through AI-driven workload distribution, leveraging machine learning and reinforcement learning techniques to enhance resource allocation efficiency. Traditional workload management methods often lead to resource underutilization, increased operational costs, and performance bottlenecks due to their static nature. In contrast, AI-based models dynamically adjust workload distribution based on real-time demand patterns, ensuring optimal resource utilization and minimizing expenses. The study evaluates the impact of AI-driven workload distribution on key performance metrics, including cost reduction, resource efficiency, latency, SLA compliance, and execution time. Experimental results indicate that AI-based scheduling reduces cloud costs by approximately 37.5%, improves resource utilization by 50%, decreases system latency by 40.9%, and enhances SLA compliance rates to 98%. The study highlights the role of predictive analytics in forecasting workload trends, enabling proactive resource allocation, and reducing energy consumption in cloud data centers. Additionally, AI-driven workload management facilitates seamless workload balancing across multi-cloud and hybrid cloud environments, promoting scalability and reducing vendor dependency. Despite challenges such as computational overhead and model interpretability, AI-powered workload distribution presents a viable solution for optimizing cloud computing operations. This research provides valuable insights into the benefits of integrating AI-driven strategies into cloud management, offering a cost-effective and sustainable approach to workload scheduling. The findings underscore the potential of AI in revolutionizing cloud computing by improving efficiency, reducing operational costs, and enhancing overall system performance. |
Keywords | AI-driven workload distribution, cloud cost optimization, machine learning in cloud computing, resource allocation efficiency, dynamic workload scheduling |
Field | Computer > Artificial Intelligence / Simulation / Virtual Reality |
Published In | Volume 7, Issue 2, March-April 2025 |
Published On | 2025-03-18 |
DOI | https://doi.org/10.36948/ijfmr.2025.v07i02.39385 |
Short DOI | https://doi.org/g89vt6 |
Share this

E-ISSN 2582-2160

CrossRef DOI is assigned to each research paper published in our journal.
IJFMR DOI prefix is
10.36948/ijfmr
Downloads
All research papers published on this website are licensed under Creative Commons Attribution-ShareAlike 4.0 International License, and all rights belong to their respective authors/researchers.
