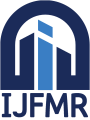
International Journal For Multidisciplinary Research
E-ISSN: 2582-2160
•
Impact Factor: 9.24
A Widely Indexed Open Access Peer Reviewed Multidisciplinary Bi-monthly Scholarly International Journal
Home
Research Paper
Submit Research Paper
Publication Guidelines
Publication Charges
Upload Documents
Track Status / Pay Fees / Download Publication Certi.
Editors & Reviewers
View All
Join as a Reviewer
Get Membership Certificate
Current Issue
Publication Archive
Conference
Publishing Conf. with IJFMR
Upcoming Conference(s) ↓
WSMCDD-2025
GSMCDD-2025
Conferences Published ↓
RBS:RH-COVID-19 (2023)
ICMRS'23
PIPRDA-2023
Contact Us
Plagiarism is checked by the leading plagiarism checker
Call for Paper
Volume 7 Issue 2
March-April 2025
Indexing Partners






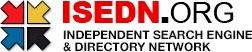

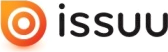


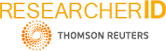
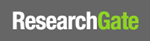
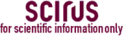
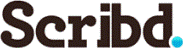

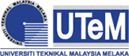
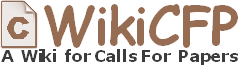
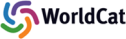
Real Time Fault Identification In Circuitry With Immediate Alert System Using Deep Learning
Author(s) | Ms. Janani J, Kalpana S, Vijayalakshmi D M |
---|---|
Country | India |
Abstract | The web application is designed for real-time fault identification in electrical circuitry, integrated with an immediate alert system, leveraging deep learning techniques with the YOLOv7 algorithm. By harnessing advanced computer vision and deep learning-based circuitry diagnostics, the system enables precise, automated detection of faults within electrical components, significantly reducing manual inspection efforts. Continuous monitoring ensures that even minor anomalies indicative of potential failures are swiftly recognized, allowing for early-stage intervention before critical malfunctions occur. Upon detecting faults, the system triggers real-time alerts through multiple channels, such as SMS, email, or dashboard notifications, ensuring prompt response by maintenance teams. This proactive approach minimizes downtime, prevents equipment failures, and enhances the safety and longevity of electrical systems. Additionally, the framework incorporates adaptive learning mechanisms, enabling it to dynamically adjust to evolving fault patterns and environmental variations. Over time, the model refines its accuracy, making it robust against new or previously unseen faults. To evaluate the system’s effectiveness, extensive experimental validation was conducted using real-world datasets, demonstrating high fault detection accuracy, superior processing speed, and robustness compared to conventional fault detection techniques. The proposed solution holds immense potential for industrial and commercial applications, particularly in smart grids, automated manufacturing units, aerospace systems, and IoT-enabled smart infrastructure. By integrating state-of-the-art deep learning with real-time monitoring, this system offers a scalable, efficient, and intelligent solution for ensuring the reliability and safety of modern electrical circuitry across diverse domains. |
Keywords | Deep learning, YOLOv7 algorithm, Circuitry diagnostics, Real-time fault identification, Immediate alert system, Computer vision |
Field | Engineering |
Published In | Volume 7, Issue 2, March-April 2025 |
Published On | 2025-03-22 |
DOI | https://doi.org/10.36948/ijfmr.2025.v07i02.39529 |
Short DOI | https://doi.org/g89v77 |
Share this

E-ISSN 2582-2160

CrossRef DOI is assigned to each research paper published in our journal.
IJFMR DOI prefix is
10.36948/ijfmr
Downloads
All research papers published on this website are licensed under Creative Commons Attribution-ShareAlike 4.0 International License, and all rights belong to their respective authors/researchers.
