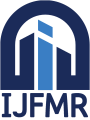
International Journal For Multidisciplinary Research
E-ISSN: 2582-2160
•
Impact Factor: 9.24
A Widely Indexed Open Access Peer Reviewed Multidisciplinary Bi-monthly Scholarly International Journal
Home
Research Paper
Submit Research Paper
Publication Guidelines
Publication Charges
Upload Documents
Track Status / Pay Fees / Download Publication Certi.
Editors & Reviewers
View All
Join as a Reviewer
Get Membership Certificate
Current Issue
Publication Archive
Conference
Publishing Conf. with IJFMR
Upcoming Conference(s) ↓
WSMCDD-2025
GSMCDD-2025
Conferences Published ↓
RBS:RH-COVID-19 (2023)
ICMRS'23
PIPRDA-2023
Contact Us
Plagiarism is checked by the leading plagiarism checker
Call for Paper
Volume 7 Issue 2
March-April 2025
Indexing Partners






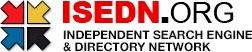

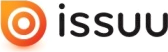


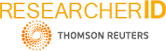
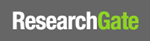
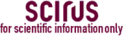
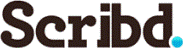

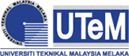
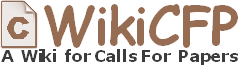
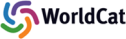
Skin Lesion Segmentation Using U-Net for Automated Dermatological Image Analysis
Author(s) | Ms. Serra Aksoy |
---|---|
Country | Germany |
Abstract | AI in dermatology has made a huge impact in the detection of skin cancer. One of the key advancements is the use of the U-Net architecture for skin lesion segmentation. U-Net as an encoder-decoder network learns low- and high-level features efficiently, ideally appropriate for biomedical image segmentation. The trained U-Net network on the ISIC 2018 dataset resulted in a Dice Similarity Coefficient of 0.89, Jaccard Index of 0.82, and the sensitivity of 91.5%, which reflects its higher ability to detect lesion boundaries. AI models are effective at classification with 92.3% accuracy, 89.7% precision, and 90.1% F1-score in benign vs. malignant lesion detection. Segmentation along with classification decreases the time for diagnosis with less human error. AI diagnostic accuracy can be equal to or even better than dermatologists in some cases. The combination of U-Net segmentation with AI classification is a critical step forward in the automatic analysis of dermatological images. With near-expert performance from these models, patient outcomes can be enhanced by early and accurate detection of skin cancer. Future efforts will be aimed at additional model generalization and clinical deployment of AI diagnostic systems. |
Keywords | Dermatology, Skin Cancer, CNN, Melanoma Classification, ISIC Dataset. |
Field | Computer > Artificial Intelligence / Simulation / Virtual Reality |
Published In | Volume 7, Issue 2, March-April 2025 |
Published On | 2025-03-24 |
DOI | https://doi.org/10.36948/ijfmr.2025.v07i02.39613 |
Short DOI | https://doi.org/g89v7j |
Share this

E-ISSN 2582-2160

CrossRef DOI is assigned to each research paper published in our journal.
IJFMR DOI prefix is
10.36948/ijfmr
Downloads
All research papers published on this website are licensed under Creative Commons Attribution-ShareAlike 4.0 International License, and all rights belong to their respective authors/researchers.
