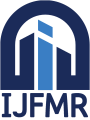
International Journal For Multidisciplinary Research
E-ISSN: 2582-2160
•
Impact Factor: 9.24
A Widely Indexed Open Access Peer Reviewed Multidisciplinary Bi-monthly Scholarly International Journal
Home
Research Paper
Submit Research Paper
Publication Guidelines
Publication Charges
Upload Documents
Track Status / Pay Fees / Download Publication Certi.
Editors & Reviewers
View All
Join as a Reviewer
Get Membership Certificate
Current Issue
Publication Archive
Conference
Publishing Conf. with IJFMR
Upcoming Conference(s) ↓
WSMCDD-2025
GSMCDD-2025
Conferences Published ↓
RBS:RH-COVID-19 (2023)
ICMRS'23
PIPRDA-2023
Contact Us
Plagiarism is checked by the leading plagiarism checker
Call for Paper
Volume 7 Issue 2
March-April 2025
Indexing Partners






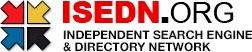

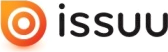


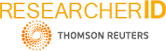
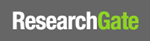
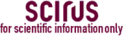
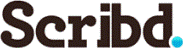

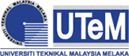
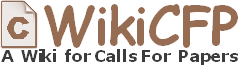
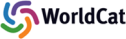
Enhancing Cyber Threat Detection Accuracy: An AI-Powered Approach with Feature Selection and Machine Learning with Ensemble Learning for Cyber Threat Detection
Author(s) | Ms. Aswani P, Soumya T, Shaji B, Justin Jose |
---|---|
Country | India |
Abstract | The rapid evolution of cyber threats necessitates advanced detection mechanisms to ensure robust network security. This study presents an AI-driven ensemble-based cyber threat detection system leveraging the CICIDS2017 dataset. Our multi-stage methodology integrates data preprocessing, attack data filtering, feature selection, and machine learning model evaluation. Data preprocessing involves cleaning, normalization, and handling missing values to enhance data quality. Attack data filtering isolates malicious and benign traffic for effective model training. Feature selection employs the Random Forest Regressor to identify key predictive attributes. The proposed system evaluates multiple machine learning algorithms, including Naive Bayes, Quadratic Discriminant Analysis (QDA), and Multi-Layer Perceptron (MLP), considering accuracy, precision, and computational efficiency. Furthermore, an ensemble model aggregates predictions from multiple classifiers to enhance detection reliability. A web-based Streamlit application facilitates real-time attack classification, presenting ensemble-based probabilistic predictions for eight attack types, including DDoS, DoS variants, and infiltration attempts. The results highlight the potential of integrating ensemble learning with feature selection and preprocessing techniques to reduce false positives, improve detection accuracy, and enable real-time threat mitigation in large-scale networks. |
Keywords | Cyber threat detection, Ensemble learning, Machine learning, Feature selection, Data preprocessing, CICIDS2017 dataset, Network traffic analysis, AI-driven cybersecurity, Real-time attack classification. |
Field | Computer > Network / Security |
Published In | Volume 7, Issue 2, March-April 2025 |
Published On | 2025-03-26 |
DOI | https://doi.org/10.36948/ijfmr.2025.v07i02.39812 |
Short DOI | https://doi.org/g892pn |
Share this

E-ISSN 2582-2160

CrossRef DOI is assigned to each research paper published in our journal.
IJFMR DOI prefix is
10.36948/ijfmr
Downloads
All research papers published on this website are licensed under Creative Commons Attribution-ShareAlike 4.0 International License, and all rights belong to their respective authors/researchers.
