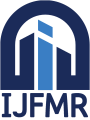
International Journal For Multidisciplinary Research
E-ISSN: 2582-2160
•
Impact Factor: 9.24
A Widely Indexed Open Access Peer Reviewed Multidisciplinary Bi-monthly Scholarly International Journal
Home
Research Paper
Submit Research Paper
Publication Guidelines
Publication Charges
Upload Documents
Track Status / Pay Fees / Download Publication Certi.
Editors & Reviewers
View All
Join as a Reviewer
Get Membership Certificate
Current Issue
Publication Archive
Conference
Publishing Conf. with IJFMR
Upcoming Conference(s) ↓
WSMCDD-2025
GSMCDD-2025
Conferences Published ↓
RBS:RH-COVID-19 (2023)
ICMRS'23
PIPRDA-2023
Contact Us
Plagiarism is checked by the leading plagiarism checker
Call for Paper
Volume 7 Issue 2
March-April 2025
Indexing Partners






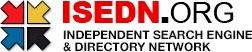

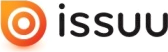


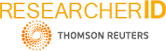
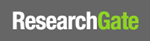
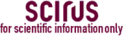
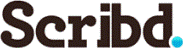

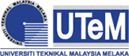
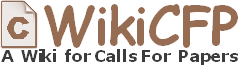
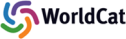
Multi-descriptor Enabled Leaf Disease Detection using Machine Learning Methods
Author(s) | C. Venkata Sudhakar, G. Umamaheswara Reddy |
---|---|
Country | India |
Abstract | India is an agricultural country. Two-thirds of the Indian populations work in agriculture, making it the backbone of the country's economic system. Leaf disease is a major challenge in agriculture that affects crop yield and quality. In recent years, advancements methods for accurately and effectively detecting leaf diseases have been developed thanks to advances in machine learning and computer vision. The objective of this work is to create a machine learning-based Random Forest Regression Algorithm for automatically detecting leaf diseases. In the suggested methodology, leaf pictures are acquired, subjected to pre-processing which includes RGB image and HSV image conversion, multi-descriptor feature extraction includes Hu moments, Haralick, color histogram and classification using Random Forest Regression Algorithm. Metrics including accuracy, precision, recall, and f1-score are used to assess the system's performance by implementing in Python 3.8. The findings of the study indicate that the proposed system achieves precision 98 %, recall 98 %, f1-score 98%, test accuracy 97.81 %, while validation accuracy is 95.93 %. The developed system has potential applications in precision agriculture, enabling farmers to detect and treat plant diseases early, thereby reducing crop losses and increasing yields. |
Keywords | Leaf Disease Detection, Random Forest regression, Python, Google Colab |
Field | Engineering |
Published In | Volume 5, Issue 3, May-June 2023 |
Published On | 2023-06-26 |
DOI | https://doi.org/10.36948/ijfmr.2023.v05i03.4008 |
Short DOI | https://doi.org/gsd5bn |
Share this

E-ISSN 2582-2160

CrossRef DOI is assigned to each research paper published in our journal.
IJFMR DOI prefix is
10.36948/ijfmr
Downloads
All research papers published on this website are licensed under Creative Commons Attribution-ShareAlike 4.0 International License, and all rights belong to their respective authors/researchers.
