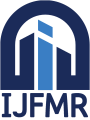
International Journal For Multidisciplinary Research
E-ISSN: 2582-2160
•
Impact Factor: 9.24
A Widely Indexed Open Access Peer Reviewed Multidisciplinary Bi-monthly Scholarly International Journal
Home
Research Paper
Submit Research Paper
Publication Guidelines
Publication Charges
Upload Documents
Track Status / Pay Fees / Download Publication Certi.
Editors & Reviewers
View All
Join as a Reviewer
Get Membership Certificate
Current Issue
Publication Archive
Conference
Publishing Conf. with IJFMR
Upcoming Conference(s) ↓
WSMCDD-2025
GSMCDD-2025
Conferences Published ↓
RBS:RH-COVID-19 (2023)
ICMRS'23
PIPRDA-2023
Contact Us
Plagiarism is checked by the leading plagiarism checker
Call for Paper
Volume 7 Issue 2
March-April 2025
Indexing Partners






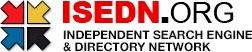

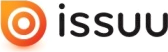


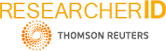
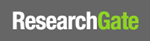
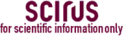
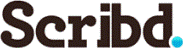

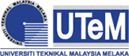
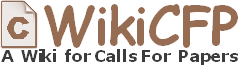
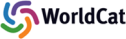
Efficientnetb0-optimized Detection:revolutionary Kidney Cachexia Nutritional Evaluation in Healthcare Innovation
Author(s) | Prof. Ms. Sumathi R, Ms. Gunasubha K, Ms. Harshini B, Ms. Badhrunnisha Z |
---|---|
Country | India |
Abstract | The identification of kidney illnesses is being revolutionized by deep learning approaches such as Convolutional Neural Networks (CNNs). CNNs are useful tools for analyzing medical imaging data, including ultrasounds and CT scans, and spotting structural anomalies in the kidneys. Conversely, CNNs may create representations of kidney-related features and model intricate data distributions, which aids in the detection of subtle illness patterns. Together, these techniques improve the precision of disease progression forecasts and individualized treatment regimens while facilitating the early detection of diseases such as tumors, stones, and chronic renal disease. Significant progress has been achieved in the early identification, diagnosis, and monitoring of kidney illnesses with the incorporation of deep learning techniques such as Convolutional Neural Networks (CNNs). CNNs are essential for the study of several imaging modalities, including MRIs, CT scans, and ultrasounds, because of their capacity to process high dimensional image data. These models are very good at differentiating between healthy and diseased tissues, identifying and segmenting kidney structures, and spotting anomalies like tumors, cysts, and stones. CNNs can automate image interpretation by identifying complex patterns in medical images, which could lower human error and improve diagnostic precision. Moreover, by integrating both structural (image-based) and functional (clinical and laboratory- based) data, the combination of CNNs in hybrid models can allow for a more thorough knowledge of kidney health. Because it can both anticipate the possibility of a disease and provide customized therapies based on individual approach to patient care. This development creates new risk factors, this synergy enables a more individualized opportunities for precision medicine, which improves patient outcomes and quality of life by tailoring treatment regimens to the particulars of each patient's renal disease. Future kidney disease detection and treatment will be more precise, quick, and individualized as these methods develop further. |
Keywords | Convolutional Neural Networks; Deep Learning; Automated Diagnosis; Hybrid Models |
Field | Engineering |
Published In | Volume 7, Issue 2, March-April 2025 |
Published On | 2025-04-05 |
DOI | https://doi.org/10.36948/ijfmr.2025.v07i02.40228 |
Short DOI | https://doi.org/g9dgxm |
Share this

E-ISSN 2582-2160

CrossRef DOI is assigned to each research paper published in our journal.
IJFMR DOI prefix is
10.36948/ijfmr
Downloads
All research papers published on this website are licensed under Creative Commons Attribution-ShareAlike 4.0 International License, and all rights belong to their respective authors/researchers.
