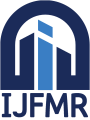
International Journal For Multidisciplinary Research
E-ISSN: 2582-2160
•
Impact Factor: 9.24
A Widely Indexed Open Access Peer Reviewed Multidisciplinary Bi-monthly Scholarly International Journal
Home
Research Paper
Submit Research Paper
Publication Guidelines
Publication Charges
Upload Documents
Track Status / Pay Fees / Download Publication Certi.
Editors & Reviewers
View All
Join as a Reviewer
Get Membership Certificate
Current Issue
Publication Archive
Conference
Publishing Conf. with IJFMR
Upcoming Conference(s) ↓
WSMCDD-2025
GSMCDD-2025
Conferences Published ↓
RBS:RH-COVID-19 (2023)
ICMRS'23
PIPRDA-2023
Contact Us
Plagiarism is checked by the leading plagiarism checker
Call for Paper
Volume 7 Issue 2
March-April 2025
Indexing Partners






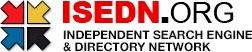

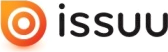


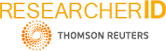
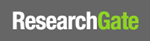
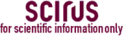
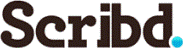

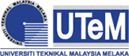
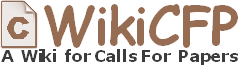
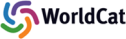
Heart Disease Prediction and Risk Analysis by Machine Learning Techniques.
Author(s) | Mr. Ninad Dilip Sarang, Omkar Singh, Amit Kumar Pandey |
---|---|
Country | India |
Abstract | Cardiovascular disease remains one of the leading causes of global mortality, underscoring the urgent need for robust early detection systems. This study introduces an innovative machine learning framework for heart disease prediction using the Cleveland dataset (sourced from the UCI repository via Kaggle). We employ a Decision Tree classifier for its transparency and visual interpretability, and benchmark its performance against a Gaussian Naïve Bayes model. Our data pipeline includes rigorous preprocessing steps—median imputation, Min-Max normalization, and stratified train-test partitioning—to ensure high-quality inputs. Visual outputs, including a detailed Decision Tree diagram (Figure 1) and a Principal Component Analysis (PCA) projection (Figure 2), elucidate the underlying data structure and decision boundaries. Although the Gaussian Naïve Bayes model demonstrates higher accuracy, the Decision Tree’s clear decision paths offer invaluable insights for clinical applications. This work balances predictive performance with interpretability, paving the way for future research with hybrid models and expanded clinical feature sets. |
Keywords | Heart disease prediction, machine learning, Decision Tree, Naïve Bayes, interpretability, risk analysis, Cleveland dataset, healthcare analytics. |
Published In | Volume 7, Issue 2, March-April 2025 |
Published On | 2025-04-07 |
DOI | https://doi.org/10.36948/ijfmr.2025.v07i02.40746 |
Short DOI | https://doi.org/g9dnbf |
Share this

E-ISSN 2582-2160

CrossRef DOI is assigned to each research paper published in our journal.
IJFMR DOI prefix is
10.36948/ijfmr
Downloads
All research papers published on this website are licensed under Creative Commons Attribution-ShareAlike 4.0 International License, and all rights belong to their respective authors/researchers.
