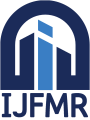
International Journal For Multidisciplinary Research
E-ISSN: 2582-2160
•
Impact Factor: 9.24
A Widely Indexed Open Access Peer Reviewed Multidisciplinary Bi-monthly Scholarly International Journal
Home
Research Paper
Submit Research Paper
Publication Guidelines
Publication Charges
Upload Documents
Track Status / Pay Fees / Download Publication Certi.
Editors & Reviewers
View All
Join as a Reviewer
Get Membership Certificate
Current Issue
Publication Archive
Conference
Publishing Conf. with IJFMR
Upcoming Conference(s) ↓
WSMCDD-2025
GSMCDD-2025
Conferences Published ↓
RBS:RH-COVID-19 (2023)
ICMRS'23
PIPRDA-2023
Contact Us
Plagiarism is checked by the leading plagiarism checker
Call for Paper
Volume 7 Issue 2
March-April 2025
Indexing Partners






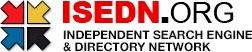

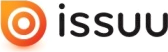


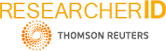
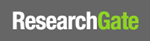
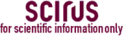
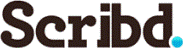

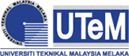
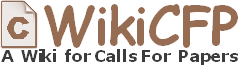
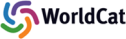
Predictive Modeling of Antibiotic Prescription Patterns Using Machine Learning in Outpatient Settings
Author(s) | Samee Mohammed, Sindhu Kommareddy, Holmes Walter |
---|---|
Country | United States |
Abstract | Antibiotic resistance is currently a critical global health issue driven by improper and excessive use of antibiotics, particularly in outpatient settings. To mitigate this, machine learning (ML) techniques hold promise by leveraging vast clinical data to predict prescription patterns and optimize the utilization of antibiotics. This study takes into account the application of ML models for predicting antibiotic prescriptions based on patient features, history, physician prescribing patterns, and diagnosing variables. Using an outpatient clinic electronic health records (EHRs) dataset, we developed and validated a range of ML models, from basic logistic regression and decision trees to random forests, support vector machines, and deep learning-based architectures. Feature selection techniques were employed to identify the most important drivers of prescription decision, such as previous use of antibiotics, comorbidities, and local antimicrobial resistance patterns. Model performance was quantified in terms of accuracy, precision, recall, and F1-score and indicated that ensemble learning methods and deep learning models offered the highest predictive accuracy. The results identify the importance of ML-based predictive analytics in supporting antibiotic stewardship programs by identifying hazardous prescription patterns and providing real-time recommendations. With such models integrated into clinical decision support systems (CDSS), clinicians can prevent improper use of antibiotics, enhance patient outcomes, and minimize antimicrobial resistance. However, issues in integration are heterogeneity of data, interpretability of predictions by ML, and ethical dilemmas to be addressed in advancing responsible implementation in healthcare settings. This research highlights the potential of ML to revolutionize antibiotic prescribing practices and impelling its future potential to enhance precision medicine and public health interventions. Improving the ML models with larger, mixed datasets and streaming real-time data needs to be considered for future research aimed at improving predictability and applicability to clinical decision-making. |
Keywords | Antibiotic prescription patterns, Machine learning, Predictive modeling, Outpatient settings, Electronic health records (EHR), Clinical decision support systems (CDSS), Antimicrobial resistance, Deep learning, Logistic regression, Random forests, Healthcare analytics, Data-driven prescribing, Feature selection, Precision medicine, Artificial intelligence in healthcare |
Field | Medical / Pharmacy |
Published In | Volume 7, Issue 2, March-April 2025 |
Published On | 2025-04-04 |
DOI | https://doi.org/10.36948/ijfmr.2025.v07i02.40879 |
Short DOI | https://doi.org/g9dg38 |
Share this

E-ISSN 2582-2160

CrossRef DOI is assigned to each research paper published in our journal.
IJFMR DOI prefix is
10.36948/ijfmr
Downloads
All research papers published on this website are licensed under Creative Commons Attribution-ShareAlike 4.0 International License, and all rights belong to their respective authors/researchers.
