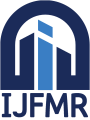
International Journal For Multidisciplinary Research
E-ISSN: 2582-2160
•
Impact Factor: 9.24
A Widely Indexed Open Access Peer Reviewed Multidisciplinary Bi-monthly Scholarly International Journal
Home
Research Paper
Submit Research Paper
Publication Guidelines
Publication Charges
Upload Documents
Track Status / Pay Fees / Download Publication Certi.
Editors & Reviewers
View All
Join as a Reviewer
Reviewer Referral Program
Get Membership Certificate
Current Issue
Publication Archive
Conference
Publishing Conf. with IJFMR
Upcoming Conference(s) ↓
WSMCDD-2025
Conferences Published ↓
RBS:RH-COVID-19 (2023)
ICMRS'23
PIPRDA-2023
Contact Us
Plagiarism is checked by the leading plagiarism checker
Call for Paper
Volume 6 Issue 4
July-August 2024
Indexing Partners






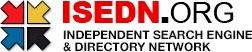

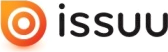


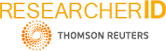
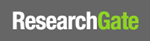
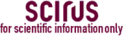
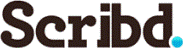

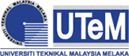
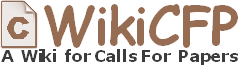
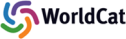
Machine Learning Methodology for Prediction of Chronic Kidney Disease
Author(s) | Miss. Divya Ravinder Pogaku, Prof. Sneha Bohra |
---|---|
Country | India |
Abstract | Chronic Kidney Disease (CKD) is a major health problem affecting millions of people worldwide. Early and accurate diagnosis of CKD is essential for successful management and treatment of the disease. In this paper, we propose a machine learning-based approach for diagnosing CKD using different classification algorithms. Our approach utilizes a combination of demographic data, medical history, and laboratory test results to predict CKD. We tested our approach using several machine learning algorithms, including decision trees, random forests, and support vector machines (SVM), and compared our results with traditional diagnostic methods. Our results show that SVM achieved the highest accuracy in diagnosing CKD, followed by decision trees and random forests. Our approach outperformed traditional diagnostic methods in terms of accuracy and reliability, demonstrating the potential of machine learning in improving CKD diagnosis. Our approach can be used to develop a computer-aided diagnosis system to assist clinicians in the early and accurate diagnosis of CKD, leading to better patient outcomes. |
Keywords | CKD, SVM,Prediction |
Field | Engineering |
Published In | Volume 5, Issue 3, May-June 2023 |
Published On | 2023-06-20 |
Cite This | Machine Learning Methodology for Prediction of Chronic Kidney Disease - Miss. Divya Ravinder Pogaku, Prof. Sneha Bohra - IJFMR Volume 5, Issue 3, May-June 2023. DOI 10.36948/ijfmr.2023.v05i03.4172 |
DOI | https://doi.org/10.36948/ijfmr.2023.v05i03.4172 |
Short DOI | https://doi.org/gsd48q |
Share this
E-ISSN 2582-2160

CrossRef DOI is assigned to each research paper published in our journal.
IJFMR DOI prefix is
10.36948/ijfmr
Downloads
All research papers published on this website are licensed under Creative Commons Attribution-ShareAlike 4.0 International License, and all rights belong to their respective authors/researchers.
