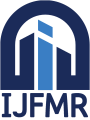
International Journal For Multidisciplinary Research
E-ISSN: 2582-2160
•
Impact Factor: 9.24
A Widely Indexed Open Access Peer Reviewed Multidisciplinary Bi-monthly Scholarly International Journal
Home
Research Paper
Submit Research Paper
Publication Guidelines
Publication Charges
Upload Documents
Track Status / Pay Fees / Download Publication Certi.
Editors & Reviewers
View All
Join as a Reviewer
Get Membership Certificate
Current Issue
Publication Archive
Conference
Publishing Conf. with IJFMR
Upcoming Conference(s) ↓
WSMCDD-2025
GSMCDD-2025
Conferences Published ↓
RBS:RH-COVID-19 (2023)
ICMRS'23
PIPRDA-2023
Contact Us
Plagiarism is checked by the leading plagiarism checker
Call for Paper
Volume 7 Issue 2
March-April 2025
Indexing Partners






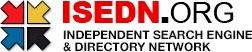

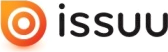


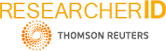
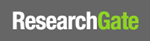
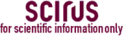
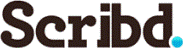

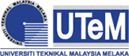
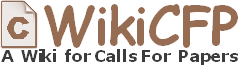
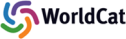
Breast Cancer Detection Using Machine Learning
Author(s) | Mr. Md. Shahbaz Hassan, Mr. Rishabh Kumar, Mr. Rohit Kumar Yadav |
---|---|
Country | India |
Abstract | Cancer mortality remains a significant challenge in developing nations, despite various preventive measures. Some cancer types still lack effective treatments. Breast cancer, one of the most prevalent forms, relies heavily on early detection for successful treatment. Accurate diagnosis plays a crucial role in managing breast cancer. Numerous studies have explored methods for predicting breast tumor types. This study utilized breast cancer tumor data from Kaggle datasets forecast tumor types. The research employed data visualization and machine learning techniques, including logistic regression and Python were used to implement these techniques and visualizations. The research aimed to conduct a comparative analysis of data visualization and machine learning applications for breast cancer detection and diagnosis. The diagnostic performance of these applications was found to be comparable in identifying breast cancers. The study demonstrated that data visualization and machine learning techniques could significantly benefit the decision-making process in cancer detection. Various machine learning and data mining techniques were proposed for breast cancer detection. The logistic regression model, incorporating all features, yielded the highest classification accuracy at 98.1%. This approach showed improved accuracy performance, suggesting potential new avenues for breast cancer detection. |
Keywords | Breast Cancer, Dataset, CNN, KNN, Naïve Bayes, Random Forest, SVM, Logistic Regression |
Field | Computer > Data / Information |
Published In | Volume 7, Issue 2, March-April 2025 |
Published On | 2025-04-19 |
DOI | https://doi.org/10.36948/ijfmr.2025.v07i02.41964 |
Short DOI | https://doi.org/g9f4zf |
Share this

E-ISSN 2582-2160

CrossRef DOI is assigned to each research paper published in our journal.
IJFMR DOI prefix is
10.36948/ijfmr
Downloads
All research papers published on this website are licensed under Creative Commons Attribution-ShareAlike 4.0 International License, and all rights belong to their respective authors/researchers.
