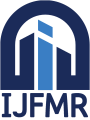
International Journal For Multidisciplinary Research
E-ISSN: 2582-2160
•
Impact Factor: 9.24
A Widely Indexed Open Access Peer Reviewed Multidisciplinary Bi-monthly Scholarly International Journal
Home
Research Paper
Submit Research Paper
Publication Guidelines
Publication Charges
Upload Documents
Track Status / Pay Fees / Download Publication Certi.
Editors & Reviewers
View All
Join as a Reviewer
Reviewer Referral Program
Get Membership Certificate
Current Issue
Publication Archive
Conference
Publishing Conf. with IJFMR
Upcoming Conference(s) ↓
WSMCDD-2025
Conferences Published ↓
RBS:RH-COVID-19 (2023)
ICMRS'23
PIPRDA-2023
Contact Us
Plagiarism is checked by the leading plagiarism checker
Call for Paper
Volume 6 Issue 4
July-August 2024
Indexing Partners






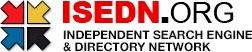

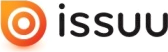


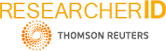
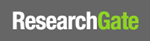
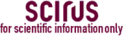
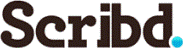

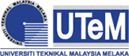
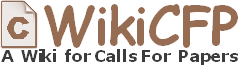
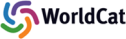
Enhancing Heart Disease Prediction Through KBEST-PCA Fusion Feature Selection and Ensemble Modeling With Gaussian Naive Bayes Boosting
Author(s) | Dhivya P, Sangavi N, Akashprabu A C, Anooskavin G |
---|---|
Country | India |
Abstract | Heart disease is a prevalent health condition with significant implications for patient health and well-being. Accurate and timely diagnosis plays a crucial role in effective treatment and management. In this study, we propose a combined approach using SelectKBest, Gaussian Naive Bayes (GNB), and Gradient Boosting Machines (GBM) to develop a robust predictive model for heart disease diagnosis. The SelectKBest algorithm is employed to identify the most informative features from the Statlog Heart Disease dataset. Statistical measures such as chi-squared test are utilized to select the top K features that exhibit the strongest associations with the target variable. The selected features are then used to train a GNB classifier, capturing the probabilistic relationships between the features and the diagnosis of heart disease. Predictions generated from the GNB model are combined with the original features, creating an extended feature matrix. Subsequently, a GBM ensemble model is trained on the extended feature matrix, leveraging the sequential combination of weak learners to improve the overall predictive performance. To evaluate the effectiveness of the proposed approach, extensive experiments are conducted on the Statlog Heart Disease dataset. Performance metrics including accuracy, precision, recall, and F1 score are used to compare the combined SelectKBest-GNB-GBM approach against individual classifiers and existing methods. |
Keywords | Heart disease diagnosis, SelectKBest, Gaussian Naive Bayes, Gradient Boosting Machines, ensemble learning, feature selection. |
Field | Medical / Pharmacy |
Published In | Volume 5, Issue 4, July-August 2023 |
Published On | 2023-07-19 |
Cite This | Enhancing Heart Disease Prediction Through KBEST-PCA Fusion Feature Selection and Ensemble Modeling With Gaussian Naive Bayes Boosting - Dhivya P, Sangavi N, Akashprabu A C, Anooskavin G - IJFMR Volume 5, Issue 4, July-August 2023. DOI 10.36948/ijfmr.2023.v05i04.4378 |
DOI | https://doi.org/10.36948/ijfmr.2023.v05i04.4378 |
Short DOI | https://doi.org/gshnbc |
Share this
E-ISSN 2582-2160

CrossRef DOI is assigned to each research paper published in our journal.
IJFMR DOI prefix is
10.36948/ijfmr
Downloads
All research papers published on this website are licensed under Creative Commons Attribution-ShareAlike 4.0 International License, and all rights belong to their respective authors/researchers.
