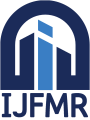
International Journal For Multidisciplinary Research
E-ISSN: 2582-2160
•
Impact Factor: 9.24
A Widely Indexed Open Access Peer Reviewed Multidisciplinary Bi-monthly Scholarly International Journal
Home
Research Paper
Submit Research Paper
Publication Guidelines
Publication Charges
Upload Documents
Track Status / Pay Fees / Download Publication Certi.
Editors & Reviewers
View All
Join as a Reviewer
Reviewer Referral Program
Get Membership Certificate
Current Issue
Publication Archive
Conference
Publishing Conf. with IJFMR
Upcoming Conference(s) ↓
WSMCDD-2025
Conferences Published ↓
RBS:RH-COVID-19 (2023)
ICMRS'23
PIPRDA-2023
Contact Us
Plagiarism is checked by the leading plagiarism checker
Call for Paper
Volume 6 Issue 4
July-August 2024
Indexing Partners






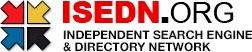

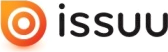


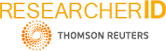
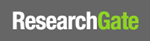
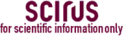
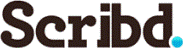

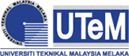
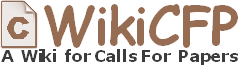
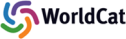
Involuntary Lung Nodules Recognition in Computed Tomography Images using 3D features Mining and Neural System
Author(s) | Arushi Bansal, Vinod Kumar Srivastava |
---|---|
Country | India |
Abstract | The best precise imaging method for determining the presence and stage of lung cancer is considered to be computed tomography (CT). On a chest X-ray, nodules are a legitimately frequent abnormality: one out of every 500 chest X-rays reveals newly diagnosed nodules. From helical CT scans, we suggested a computer-aided diagnostic (CAD) method to detect small-size lung knots, which range in size from 1 mm to 6 mm. An insignificant, curving (parenchymal knot) or caterpillar-shaped (juxta pleural nodule) wound in the lungs is known as a pulmonic knot. Because they each have a higher radio-density than the lung parenchyma, they appear snowy in images. Lung knots may indicate a lung cancer, and identifying them early on improves patient survival rates. The best precise imaging technique for finding knots is thought to be CT. However, because there is so much data in each study, analysis becomes difficult. This suggests that a human radiologist could have missed a knot. The proposed CAD method aims to reduce omissions and shorten the time needed for radiologist review of the picture. Our method classifies nodule items from non-nodule objects using a three-layer Feed Forward Neural Network with directed knowledge based on back-propagation technique and CLAHE, an alternative to Histogram Equalization that reduces the noise amplification. The technique was tested on Windows after being built in Matlab. Simple graphic user interface is supplied for convenient control. |
Keywords | Computer Aided Diagnosis, Computed Tomography, Contrast Limited Adaptive Histogram Equalization |
Field | Engineering |
Published In | Volume 5, Issue 4, July-August 2023 |
Published On | 2023-07-15 |
Cite This | Involuntary Lung Nodules Recognition in Computed Tomography Images using 3D features Mining and Neural System - Arushi Bansal, Vinod Kumar Srivastava - IJFMR Volume 5, Issue 4, July-August 2023. DOI 10.36948/ijfmr.2023.v05i04.4424 |
DOI | https://doi.org/10.36948/ijfmr.2023.v05i04.4424 |
Short DOI | https://doi.org/gshm88 |
Share this
E-ISSN 2582-2160

CrossRef DOI is assigned to each research paper published in our journal.
IJFMR DOI prefix is
10.36948/ijfmr
Downloads
All research papers published on this website are licensed under Creative Commons Attribution-ShareAlike 4.0 International License, and all rights belong to their respective authors/researchers.
