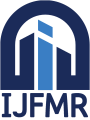
International Journal For Multidisciplinary Research
E-ISSN: 2582-2160
•
Impact Factor: 9.24
A Widely Indexed Open Access Peer Reviewed Multidisciplinary Bi-monthly Scholarly International Journal
Home
Research Paper
Submit Research Paper
Publication Guidelines
Publication Charges
Upload Documents
Track Status / Pay Fees / Download Publication Certi.
Editors & Reviewers
View All
Join as a Reviewer
Reviewer Referral Program
Get Membership Certificate
Current Issue
Publication Archive
Conference
Publishing Conf. with IJFMR
Upcoming Conference(s) ↓
WSMCDD-2025
Conferences Published ↓
RBS:RH-COVID-19 (2023)
ICMRS'23
PIPRDA-2023
Contact Us
Plagiarism is checked by the leading plagiarism checker
Call for Paper
Volume 6 Issue 4
July-August 2024
Indexing Partners






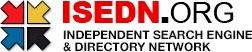

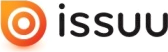


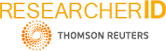
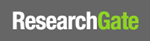
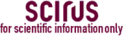
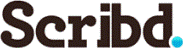

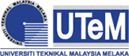
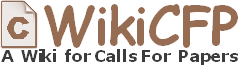
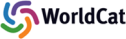
Heart Disease Prediction using Hybrid Machine Learning
Author(s) | SHAKIR ALI, Mohammad Saifuddin, Pankaj Mishra |
---|---|
Country | India |
Abstract | heart disease usually refers to conditions such as narrowed or blocked blood vessels leading to heart failure, pain due to decreased blood flow to the heart (angina), or stroke. Heart disease is one of the leading causes of mortality in the world today. It contributes to about 30% of all deaths worldwide. Predicting cardiovascular disease is a critical challenge in the field of clinical data analytics. Machine learning (ML) has been shown to be effective in making decisions and predictions from the large amount of data generated in the healthcare industry. Machine learning is being used in many fields around the world. The healthcare industry is not exempt. Machine learning can play a significant role in predicting musculoskeletal conditions, heart disease and more. Such information, when predicted in a timely manner, can provide physicians with important clues so they can tailor their diagnosis and treatment to each patient. We are working on predicting potential heart disease in humans using machine learning algorithms. In this work, we present a novel method for identifying important variables that improve the precision of “cardiovascular disease” prediction. Along with a number of feature combinations and well-known classification techniques, the "prediction" model is introduced. When preprocessing the data in an efficient way we can achieve better performance. Preprocessing means handling missing values, removing outliers, balancing the data, scaling the feature, and optimizing the hyperparameter for the tree, selecting the important feature using the feature score technique. Experiment conducted shows that preprocessing technique helps us to get better performances. The experiment result also shows that hybrid random forest with linear model and feature score (HRFLMFS) prediction model for heart disease gives us a better result. |
Keywords | Cleveland Heart Disease Database, Decision Trees, Random Forest, Hybrid algorithm, Machine learning |
Field | Engineering |
Published In | Volume 5, Issue 4, July-August 2023 |
Published On | 2023-07-29 |
Cite This | Heart Disease Prediction using Hybrid Machine Learning - SHAKIR ALI, Mohammad Saifuddin, Pankaj Mishra - IJFMR Volume 5, Issue 4, July-August 2023. DOI 10.36948/ijfmr.2023.v05i04.4705 |
DOI | https://doi.org/10.36948/ijfmr.2023.v05i04.4705 |
Short DOI | https://doi.org/gsjjqs |
Share this
E-ISSN 2582-2160

CrossRef DOI is assigned to each research paper published in our journal.
IJFMR DOI prefix is
10.36948/ijfmr
Downloads
All research papers published on this website are licensed under Creative Commons Attribution-ShareAlike 4.0 International License, and all rights belong to their respective authors/researchers.
