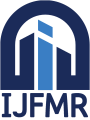
International Journal For Multidisciplinary Research
E-ISSN: 2582-2160
•
Impact Factor: 9.24
A Widely Indexed Open Access Peer Reviewed Multidisciplinary Bi-monthly Scholarly International Journal
Home
Research Paper
Submit Research Paper
Publication Guidelines
Publication Charges
Upload Documents
Track Status / Pay Fees / Download Publication Certi.
Editors & Reviewers
View All
Join as a Reviewer
Get Membership Certificate
Current Issue
Publication Archive
Conference
Publishing Conf. with IJFMR
Upcoming Conference(s) ↓
WSMCDD-2025
GSMCDD-2025
AIMAR-2025
Conferences Published ↓
ICCE (2025)
RBS:RH-COVID-19 (2023)
ICMRS'23
PIPRDA-2023
Contact Us
Plagiarism is checked by the leading plagiarism checker
Call for Paper
Volume 7 Issue 4
July-August 2025
Indexing Partners






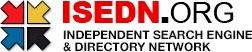

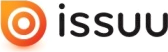


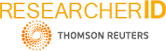
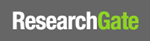
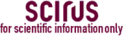
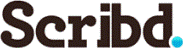

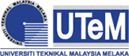
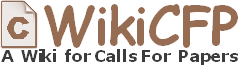
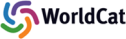
DeepCredNet: Empowering Credibility Detection in Online Media Posts with Robust Deep Neural Networks
Author(s) | Bheema Shanker Neyigapula, Praveen Babu G |
---|---|
Country | India |
Abstract | The rampant dissemination of misinformation and fake news through online media necessitates effective methods for detecting credibility. This study proposes a novel approach that employs a deep neural network (DNN) architecture, specifically Long Short-Term Memory (LSTM), to accurately assess the credibility of online media posts. The proposed LSTM-based DNN model leverages the inherent sequential nature of textual content and metadata associated with the posts. By capturing long-term dependencies and temporal dynamics, the model effectively learns intricate patterns and features crucial for credibility detection. A large labelled dataset comprising both credible and non-credible posts is employed to train the LSTM-based DNN. The input features encompass textual content, user information, and contextual details such as the post source and timing. The LSTM layers within the network enable the model to capture and retain relevant information over extended periods, enhancing its discriminative capabilities. Experimental evaluations validate the efficacy of the proposed approach, showcasing its ability to discern between credible and non-credible online media posts with high accuracy and robust performance. The real-time applicability of this method enables prompt credibility assessment, offering valuable support in combating misinformation and aiding users in making informed decisions while consuming online media. |
Keywords | credibility detection, online media posts, deep neural networks, LSTM, trustworthiness, data preprocessing, training, evaluation, user trust, online information consumption, social media platforms, content verification tools, real-time assessments |
Field | Computer > Artificial Intelligence / Simulation / Virtual Reality |
Published In | Volume 5, Issue 4, July-August 2023 |
Published On | 2023-08-15 |
DOI | https://doi.org/10.36948/ijfmr.2023.v05i04.5374 |
Short DOI | https://doi.org/gsmnph |
Share this

E-ISSN 2582-2160

CrossRef DOI is assigned to each research paper published in our journal.
IJFMR DOI prefix is
10.36948/ijfmr
Downloads
All research papers published on this website are licensed under Creative Commons Attribution-ShareAlike 4.0 International License, and all rights belong to their respective authors/researchers.
