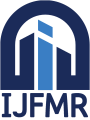
International Journal For Multidisciplinary Research
E-ISSN: 2582-2160
•
Impact Factor: 9.24
A Widely Indexed Open Access Peer Reviewed Multidisciplinary Bi-monthly Scholarly International Journal
Home
Research Paper
Submit Research Paper
Publication Guidelines
Publication Charges
Upload Documents
Track Status / Pay Fees / Download Publication Certi.
Editors & Reviewers
View All
Join as a Reviewer
Reviewer Referral Program
Get Membership Certificate
Current Issue
Publication Archive
Conference
Publishing Conf. with IJFMR
Upcoming Conference(s) ↓
WSMCDD-2025
Conferences Published ↓
RBS:RH-COVID-19 (2023)
ICMRS'23
PIPRDA-2023
Contact Us
Plagiarism is checked by the leading plagiarism checker
Call for Paper
Volume 6 Issue 4
July-August 2024
Indexing Partners






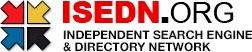

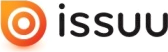


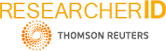
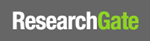
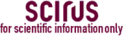
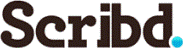

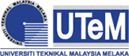
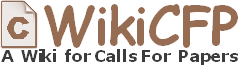
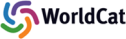
Sentiment Analysis in Online Product Reviews: Mining Customer Opinions for Sentiment Classification
Author(s) | Lakshay Bharadwaj |
---|---|
Country | India |
Abstract | Online product reviews have become a valuable resource for consumers seeking detailed information and making informed choices. The process of automatically extracting sentiment or opinions from these reviews heavily relies on sentiment analysis, a branch of Natural Language Processing (NLP). This research article focuses on sentiment categorization in online product evaluations, utilizing innovative techniques for mining consumer opinions. The project aims to establish a robust framework for sentiment analysis that accurately classifies emotions expressed in these reviews. The proposed system incorporates advanced deep learning and machine learning methods to enhance data classification and extract fine-grained sentiment information. The study addresses the unique challenges of sentiment analysis in the context of online product evaluations, including polarity changes, sarcasm, and domain-specific sentiment expressions, which often pose significant obstacles to precise sentiment classification. The approach combines feature engineering and deep learning techniques, extracting lexical, syntactic, and semantic features such as part-of-speech tags, n-grams, sentiment lexicons, and word embeddings from the review texts. Convolutional neural networks (CNNs) and recurrent neural networks (RNNs) are employed as sophisticated neural network architectures to leverage these features, creating robust representations and capturing contextual information. The suggested architecture is extensively evaluated on a large dataset of online product reviews, demonstrating superior performance in sentiment categorization compared to existing approaches. The evaluation encompasses various sentiment classes, measuring metrics like accuracy, recall, and F1-score, and assessing the framework's adaptability to different product domains. The study showcases the effectiveness of advanced machine learning and deep learning algorithms in sentiment categorization, advancing the field of sentiment analysis for online product evaluations. Businesses can gain valuable insights into customer sentiment and make well-informed decisions regarding product enhancements and marketing strategies by leveraging the proposed framework |
Keywords | Sentiment analysis, N-grams, Neural network architectures, Convolutional neural networks (CNNs), Recurrent neural networks (RNNs), F1-score. |
Field | Computer > Artificial Intelligence / Simulation / Virtual Reality |
Published In | Volume 5, Issue 5, September-October 2023 |
Published On | 2023-09-03 |
Cite This | Sentiment Analysis in Online Product Reviews: Mining Customer Opinions for Sentiment Classification - Lakshay Bharadwaj - IJFMR Volume 5, Issue 5, September-October 2023. DOI 10.36948/ijfmr.2023.v05i05.6090 |
DOI | https://doi.org/10.36948/ijfmr.2023.v05i05.6090 |
Short DOI | https://doi.org/gsn748 |
Share this
E-ISSN 2582-2160

CrossRef DOI is assigned to each research paper published in our journal.
IJFMR DOI prefix is
10.36948/ijfmr
Downloads
All research papers published on this website are licensed under Creative Commons Attribution-ShareAlike 4.0 International License, and all rights belong to their respective authors/researchers.
