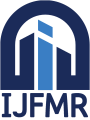
International Journal For Multidisciplinary Research
E-ISSN: 2582-2160
•
Impact Factor: 9.24
A Widely Indexed Open Access Peer Reviewed Multidisciplinary Bi-monthly Scholarly International Journal
Home
Research Paper
Submit Research Paper
Publication Guidelines
Publication Charges
Upload Documents
Track Status / Pay Fees / Download Publication Certi.
Editors & Reviewers
View All
Join as a Reviewer
Get Membership Certificate
Current Issue
Publication Archive
Conference
Publishing Conf. with IJFMR
Upcoming Conference(s) ↓
WSMCDD-2025
GSMCDD-2025
Conferences Published ↓
RBS:RH-COVID-19 (2023)
ICMRS'23
PIPRDA-2023
Contact Us
Plagiarism is checked by the leading plagiarism checker
Call for Paper
Volume 7 Issue 2
March-April 2025
Indexing Partners






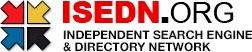

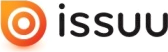


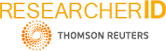
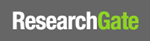
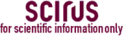
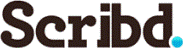

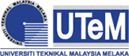
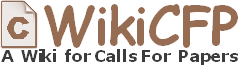
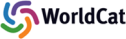
Mitigating Attrition: Data-Driven Approach Using Machine Learning and Data Engineering
Author(s) | Naveen Edapurath Vijayan |
---|---|
Country | USA |
Abstract | This paper presents a novel data-driven approach to mitigating employee attrition using machine learning and data engineering techniques. The proposed framework integrates data from various human resources systems and leverages advanced feature engineering to capture a comprehensive set of factors influencing attrition. The study outlines a robust modeling approach that addresses challenges such as imbalanced datasets, categorical data handling, and model interpretation. The methodology includes careful consideration of training and testing strategies, baseline model establishment, and the development of calibrated predictive models. The research emphasizes the importance of model interpretation using techniques like SHAP values to provide actionable insights for organizations. Key design choices in algorithm selection, hyperparameter tuning, and probability calibration are discussed. This approach enables organizations to proactively identify attrition risks and develop targeted retention strategies, ultimately reducing costs associated with employee turnover and maintaining a competitive edge in talent management. |
Keywords | Employee attrition, Machine learning, Data engineering, Predictive modeling, Feature engineering, Human resources analytics, Talent retention, Workforce analytics, Attrition prediction, Data-driven HR, SHAP values, Model interpretation, Imbalanced datasets, Probability calibration, Hyperparameter tuning, LightGBM, Proactive talent management, HR information systems, Employee sentiment analysis, Organizational performance metrics. |
Published In | Volume 1, Issue 1, July-August 2019 |
Published On | 2019-08-21 |
Share this

E-ISSN 2582-2160

CrossRef DOI is assigned to each research paper published in our journal.
IJFMR DOI prefix is
10.36948/ijfmr
Downloads
All research papers published on this website are licensed under Creative Commons Attribution-ShareAlike 4.0 International License, and all rights belong to their respective authors/researchers.
