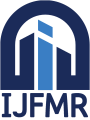
International Journal For Multidisciplinary Research
E-ISSN: 2582-2160
•
Impact Factor: 9.24
A Widely Indexed Open Access Peer Reviewed Multidisciplinary Bi-monthly Scholarly International Journal
Home
Research Paper
Submit Research Paper
Publication Guidelines
Publication Charges
Upload Documents
Track Status / Pay Fees / Download Publication Certi.
Editors & Reviewers
View All
Join as a Reviewer
Reviewer Referral Program
Get Membership Certificate
Current Issue
Publication Archive
Conference
Publishing Conf. with IJFMR
Upcoming Conference(s) ↓
WSMCDD-2025
GSMCDD-2025
Conferences Published ↓
RBS:RH-COVID-19 (2023)
ICMRS'23
PIPRDA-2023
Contact Us
Plagiarism is checked by the leading plagiarism checker
Call for Paper
Volume 6 Issue 6
November-December 2024
Indexing Partners






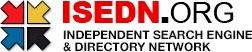

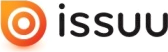


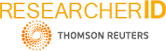
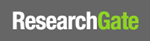
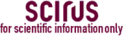
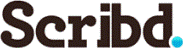

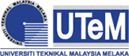
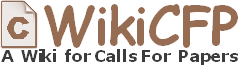
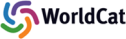
Chronic Kidney Disease Prediction Using Machine Learning
Author(s) | O.Nikhilesh Reddy, K SAI GOWTHAM, DVM KARTHIK, SHAIK ABDUL SAMI |
---|---|
Country | India |
Abstract | In today's era everyone is trying to be conscious about health although due to workload and busy schedule one gives attention to health when it shows any symptoms of some kind. However, CKD is a disease that either exhibits no symptoms at all or exhibits no signs that are particular to the condition, making it difficult to forecast, identify, and prevent such a disease and this could be led to permanently health damage, but machine learning can be hope in this problem it is best in prediction and analysis. We will employ several machine learning approaches, such as Decision Tree, KNN, Random Forest, SVM, Naive Bayes, using data that contains 24 health related attributes like age, blood pressure, sugar, glucose, taken in 2-month period of 400 patients in which 11 numeric and 14 nominal attributes in which it consists of class label named ‘Class’ which classifies patients having disease and not present. To build a model with maximum accuracy of predicting whether CKD or not and if yes then its Severity. |
Keywords | Chronic, preprocessing, Accuracy, Feature selection ,Decision Tree, KNN, SVM, Naïve- Bayes, Random Forest, Cross validation, Early Detection, Model Evaluation |
Field | Computer > Data / Information |
Published In | Volume 5, Issue 5, September-October 2023 |
Published On | 2023-09-20 |
Cite This | Chronic Kidney Disease Prediction Using Machine Learning - O.Nikhilesh Reddy, K SAI GOWTHAM, DVM KARTHIK, SHAIK ABDUL SAMI - IJFMR Volume 5, Issue 5, September-October 2023. DOI 10.36948/ijfmr.2023.v05i05.6459 |
DOI | https://doi.org/10.36948/ijfmr.2023.v05i05.6459 |
Short DOI | https://doi.org/gssfqd |
Share this

E-ISSN 2582-2160

CrossRef DOI is assigned to each research paper published in our journal.
IJFMR DOI prefix is
10.36948/ijfmr
Downloads
All research papers published on this website are licensed under Creative Commons Attribution-ShareAlike 4.0 International License, and all rights belong to their respective authors/researchers.
