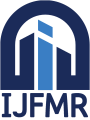
International Journal For Multidisciplinary Research
E-ISSN: 2582-2160
•
Impact Factor: 9.24
A Widely Indexed Open Access Peer Reviewed Multidisciplinary Bi-monthly Scholarly International Journal
Home
Research Paper
Submit Research Paper
Publication Guidelines
Publication Charges
Upload Documents
Track Status / Pay Fees / Download Publication Certi.
Editors & Reviewers
View All
Join as a Reviewer
Get Membership Certificate
Current Issue
Publication Archive
Conference
Publishing Conf. with IJFMR
Upcoming Conference(s) ↓
WSMCDD-2025
GSMCDD-2025
AIMAR-2025
Conferences Published ↓
ICCE (2025)
RBS:RH-COVID-19 (2023)
ICMRS'23
PIPRDA-2023
Contact Us
Plagiarism is checked by the leading plagiarism checker
Call for Paper
Volume 7 Issue 4
July-August 2025
Indexing Partners






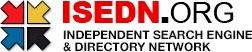

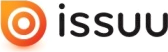


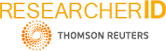
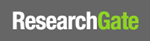
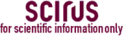
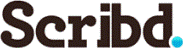

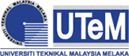
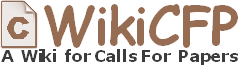
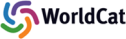
Vulnerabilities And Ethical Implications In Machine Learning
Author(s) | Shashank Walke, Sumeet Khillare |
---|---|
Country | India |
Abstract | Recent times have witnessed a convergence of expansive datasets, cost-effective parallelized computational capabilities, and progress in statistical learning techniques, particularly deep learning. This convergence has significantly propelled the integration of machine learning (ML) into commonplace applications. Machine learning models have proven their utility across diverse contexts, spanning from visual recognition tasks to personalized recommendation systems and the analysis of human language. Despite their widespread employment, the exact nature of more complex models as well as the details of their decision-making processes elude the understanding of much of the technical community. Such systems contain nebulous vulnerabilities that need to be better understood and guarded against, especially in critical applications like autonomous vehicle navigation. Recent research has elucidated some of these threats against ML systems, known as "adversarial attacks," and has attempted to describe mechanisms for both attack and defense. Within this document, we elucidate ongoing investigations, showcase tangible instances of hostile interventions, juxtapose various approaches for crafting disruptive instances, and finally delve into the ethical ramifications stemming from these susceptibilities in ML frameworks. We conclude that certain defensive measures, namely adversarial training, should be employed when creating production ready ML models. |
Keywords | Adversarial training, machine learning, vulnerabilities in ML model |
Field | Computer |
Published In | Volume 5, Issue 5, September-October 2023 |
Published On | 2023-09-17 |
DOI | https://doi.org/10.36948/ijfmr.2023.v05i05.6516 |
Short DOI | https://doi.org/gssfpz |
Share this

E-ISSN 2582-2160

CrossRef DOI is assigned to each research paper published in our journal.
IJFMR DOI prefix is
10.36948/ijfmr
Downloads
All research papers published on this website are licensed under Creative Commons Attribution-ShareAlike 4.0 International License, and all rights belong to their respective authors/researchers.
