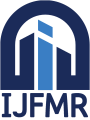
International Journal For Multidisciplinary Research
E-ISSN: 2582-2160
•
Impact Factor: 9.24
A Widely Indexed Open Access Peer Reviewed Multidisciplinary Bi-monthly Scholarly International Journal
Home
Research Paper
Submit Research Paper
Publication Guidelines
Publication Charges
Upload Documents
Track Status / Pay Fees / Download Publication Certi.
Editors & Reviewers
View All
Join as a Reviewer
Reviewer Referral Program
Get Membership Certificate
Current Issue
Publication Archive
Conference
Publishing Conf. with IJFMR
Upcoming Conference(s) ↓
WSMCDD-2025
Conferences Published ↓
RBS:RH-COVID-19 (2023)
ICMRS'23
PIPRDA-2023
Contact Us
Plagiarism is checked by the leading plagiarism checker
Call for Paper
Volume 6 Issue 4
July-August 2024
Indexing Partners






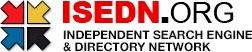

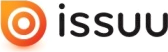


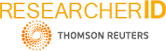
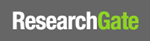
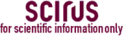
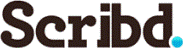

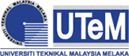
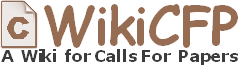
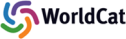
A Machine Learning-Based Risk Assessment System Prediction Algorithm for Examining Medical Insurance Costs
Author(s) | SATHWIK RAO NADIPELLI, NANTHITHA VIJAYAN, SAYALI SHELKE, DEEPTI AGRAWAL, ANIL YADAV |
---|---|
Country | India |
Abstract | Insurance is vital in today's society because it provides a critical financial safety net for individuals, families, organizations, and even governments. Based to the Global Insurance Market Analysis report, the global life insurance market was worth more than $3 trillion in 2019. It is critical in limiting the financial risks associated with unanticipated catastrophes, ensuring stability and peace of mind. In our Study, we embarked on an ambitious project to use machine learning to anticipate medical insurance expenses. Our mission began with a large dataset containing the health and demographic information of over 18,000 people, methodically obtained from Kaggle. This dataset included a plethora of variables such as age, gender, BMI, number of children, area, and, most importantly, medical charges. Our adventure progressed through numerous crucial stages. It all began with an essential component of uploading the dataset to Google Colab, which set the tone for the computational magic to follow. Preprocessing followed suit, with us dealing with missing data and unnecessary columns. To fill these gaps, we used a variety of strategies, including imputing missing values with averages or the most frequent values. In our pursuit of an optimum dataset, we meticulously changed discrete variables to continuous variables. We separated our dataset into separate subsets for training and testing using a 66:34 split ratio and used 5-fold cross-validation during the primary data analysis to enable model evaluation. Meticulous examination and citations to research publications led the important juncture of model selection. Our regression model lineup included Neural Networks, AdaBoost, Random Forest, and Gradient Boosting. The results were clear-cut, with the Neural Network coming out on top in terms of predicted performance. AdaBoost, Random Forest, and Gradient Boosting were close behind. We experimented with data visualization to better comprehend the data and the performance of the models. Sieve diagrams, bar plots, and line graphs shed light on the complexities of the dataset and the predictions of our models. Our future goal includes improving the application's accuracy and user interface, as well as assuring accessibility across all age groups. Furthermore, Our Study represents the promise as well as the potential of machine learning in the field of healthcare finance, revealing insights that have the potential to transform insurance cost estimation. |
Keywords | Insurance, Health Sector, Data Visualization, Machine learning, Regression models, Cost prediction |
Field | Computer > Artificial Intelligence / Simulation / Virtual Reality |
Published In | Volume 5, Issue 5, September-October 2023 |
Published On | 2023-09-26 |
Cite This | A Machine Learning-Based Risk Assessment System Prediction Algorithm for Examining Medical Insurance Costs - SATHWIK RAO NADIPELLI, NANTHITHA VIJAYAN, SAYALI SHELKE, DEEPTI AGRAWAL, ANIL YADAV - IJFMR Volume 5, Issue 5, September-October 2023. DOI 10.36948/ijfmr.2023.v05i05.6904 |
DOI | https://doi.org/10.36948/ijfmr.2023.v05i05.6904 |
Short DOI | https://doi.org/gstc99 |
Share this
E-ISSN 2582-2160

CrossRef DOI is assigned to each research paper published in our journal.
IJFMR DOI prefix is
10.36948/ijfmr
Downloads
All research papers published on this website are licensed under Creative Commons Attribution-ShareAlike 4.0 International License, and all rights belong to their respective authors/researchers.
