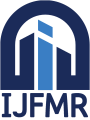
International Journal For Multidisciplinary Research
E-ISSN: 2582-2160
•
Impact Factor: 9.24
A Widely Indexed Open Access Peer Reviewed Multidisciplinary Bi-monthly Scholarly International Journal
Home
Research Paper
Submit Research Paper
Publication Guidelines
Publication Charges
Upload Documents
Track Status / Pay Fees / Download Publication Certi.
Editors & Reviewers
View All
Join as a Reviewer
Get Membership Certificate
Current Issue
Publication Archive
Conference
Publishing Conf. with IJFMR
Upcoming Conference(s) ↓
WSMCDD-2025
GSMCDD-2025
Conferences Published ↓
RBS:RH-COVID-19 (2023)
ICMRS'23
PIPRDA-2023
Contact Us
Plagiarism is checked by the leading plagiarism checker
Call for Paper
Volume 7 Issue 2
March-April 2025
Indexing Partners






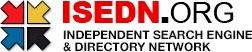

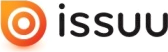


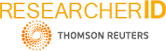
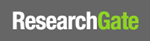
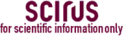
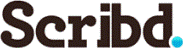

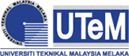
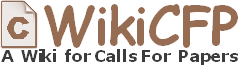
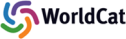
Face Mask Image Classification Using Fine-Tuning and The Effect of FGSM And PGD Attacks.
Author(s) | Kouassi Joshua Caleb Micah, Lou Qiong |
---|---|
Country | China |
Abstract | This research seeks to use a fine-tuning method for automatic diagnostics and classification of face masks without face mask images. We trained Deep Convolutional Neural Network models on the face mask dataset obtained from the Mendeley data repository to determine whether individuals will adhere to policies that face mask reduces the spread of SARS-COV-2. The proposed architectures used in this study include the VGG19, MobileNetV3, and InceptionV3 models. These models are known for their role in classifying images. They were trained using the fine-tuning approach and their respective outputs were compared. After training the face mask dataset, it can be said that the fine-tuned InceptionV3 model performed extremely well against the other models obtaining an accuracy of 99.21%, and was able to predict 99.63% of the test dataset. However, the robustness of the fine-tuned model was tested against fast gradient sign method (FGSM) and projected gradient descent (PGD) attacks which generate adversarial images using the gradients of the model. Additionally, the classification report shows after the FGSM attack that the model's accuracy was reduced by 43%. Also, after the PDG attack, the accuracy of the model was reduced by 19%. We assessed the models using many performance metrics such as precision, recall, F1 score, and accuracy before subjecting them to two common adversarial attack techniques: FGSM and PDG. Finally, we demonstrated how the proposed robust method improved the model’s defense against adversarial attacks. The findings emphasize the critical need to increase awareness about adversarial assaults on SARS-COV-2 monitoring systems and to advocate for proactive steps to protect healthcare systems from comparable threats prior to practical deployment. |
Keywords | Convolutional Neural Network, Face Mask, Fine-Tuning, FGSM attack, PDG attack |
Field | Computer > Artificial Intelligence / Simulation / Virtual Reality |
Published In | Volume 5, Issue 5, September-October 2023 |
Published On | 2023-10-02 |
DOI | https://doi.org/10.36948/ijfmr.2023.v05i05.7029 |
Short DOI | https://doi.org/gstc8d |
Share this

E-ISSN 2582-2160

CrossRef DOI is assigned to each research paper published in our journal.
IJFMR DOI prefix is
10.36948/ijfmr
Downloads
All research papers published on this website are licensed under Creative Commons Attribution-ShareAlike 4.0 International License, and all rights belong to their respective authors/researchers.
